What Does Innovation Today Tell Us About the US Economy Tomorrow?
Above all, that the nation needs to get a lot better at linking scientific advance to economically and socially valuable technologies.
How does the future of technological innovation look for the United States economy? Experts disagree. Techno-optimists such as Erik Brynjolfsson, Andrew McAfee, Martin Ford, and Ray Kurzweil claim that there are endless opportunities arising from continuing advances in computing power, artificial intelligence, and other areas of science, and that the main challenge for policy makers is to prevent mass unemployment in the face of rapid and disruptive future technological change. Pessimists such as Robert Gordon and Tyler Cowen point to slowing productivity growth and rising health and education costs as evidence that the future contributions of innovation to the economy may be weak, and that policies will be needed to promote faster growth. Is there a way to objectively address this disagreement?
Using data on innovations by start-up companies and research activities by universities, I have analyzed the types of innovations that have recently been successfully commercialized in order to identify the parts of the US innovation system that are working well and those that are not. In doing so, I distinguish between two long-term processes of technology change from which new products and services become economically feasible. The predominant viewpoint among innovation analysts is that advances in science (that is, new explanations for physical or artificial phenomena) form the basis of new product concepts and facilitate improvements in the performance and cost of the resulting technologies. Sometimes called the linear model of innovation, here I will call it the science-based process of technology change. In a second process, rapid improvements in existing technologies—such as integrated circuits, displays, smartphones, and Internet speed and cost—enable new forms of higher-level products and services to emerge; I call this the Silicon Valley process of technology change. It is largely ignored by the academic literature on innovation.
In the science-based innovation process, basic research illuminates new explanations and applied research uses these explanations to improve the performance and cost of science-based technologies such as carbon nanotubes, superconductors, quantum dot solar cells, and organic transistors. In the Silicon Valley process, the emergence of e-commerce, social networking, smartphones, tablet computers, and ride sharing did not directly depend on advances in science, nor did improvements in their overall design. Instead, the performance and cost of these technologies became economically feasible through continual, generally incremental improvements in technological performance.
In making the distinction between these two different processes of technological innovation, I acknowledge that advances in science were indirectly necessary for new forms of products and services to emerge from the Silicon Valley process of technology change. These advances enabled rapid improvements in integrated circuits, magnetic storage, fiber optics, lasers, light-emitting diodes (LEDs), and lithium ion batteries. Without these advances, improvements would have been slower and fewer electronic products, computers, and Internet services and content would have emerged. The distinction is useful, however, because it helps to better understand the types of innovations that have recently been successfully commercialized, and thus which parts of the US innovation system are working better than others. Such understanding, as we shall see, has useful implications for rethinking policies that are mostly informed by a science-based understanding of innovation.
The science-based process of technology change
The predominant view of the sources of innovation is that advances in science—new explanations of natural or artificial phenomena—play a key role in economic growth because they facilitate the creation and demonstration of new concepts and inventions. New explanations of physical or artificial phenomena such as PN junctions, optical amplification, electro-luminescence, photovoltaics, and light modulation emerged from basic research and formed the basis for new concepts such as transistors, lasers, LEDs, solar cells, and liquid crystal displays, respectively. Older examples of science-based technologies include vacuum tubes and radio and television, and more recent examples include biotech products and the technologies that I discuss below. Biotechnology depends a great deal on advances in science because a better understanding of both human biology and drug design are needed for drugs to provide value.
Advances in science can also facilitate rapid improvements in the cost and performance of new technologies, including pre-commercialization improvements, because they help engineers and scientists find better product and process designs. The early pre-commercialization improvements are typically classified as applied research and the subsequent ones are typically classified as development, where advances in science play an important role in identifying the new product and process designs in both applied research and development. My study of 13 science-based technologies, published in Research Policy in 2015, found that one key design change was the creation of new materials that better exploit relevant physical phenomena. New materials enabled most of the rapid improvements in the performance and cost of organic transistors, solar cells, and displays; of quantum dot solar cells and displays; and of quantum computers. This is because the new materials better exploited the physical phenomena for which these technologies and their concepts depended. Advances in science helped scientists and engineers search for, identify, and create these new materials because the advances illuminated the relevant physical phenomena.
For new forms of integrated circuits such as superconducting Josephson junctions and resistive RAM (random access memory), reductions in the scale of specific dimensions enabled most of the improvements, and these reductions were facilitated by advances in science. Most people are familiar with the reductions in scale that enable Moore’s Law (the observation that the number of transistors on a microprocessor doubles every 18 to 24 months). Just as conventional integrated circuits such as microprocessors and memory benefit from reductions in the scale of transistors and memory cells, respectively, resistive RAM benefits from smaller memory cells and superconducting Josephson junctions benefit from reductions in the scale of their active elements. And finding new designs that have smaller scale is facilitated by advances in science that help designers understand the various design trade-offs that emerge as dimensions are made smaller.
These types of examples demonstrate how advances in science can both facilitate improvements in new technologies and enable new concepts, and they are one reason science receives large support from federal, state, and local governments. Universities are the largest beneficiaries of this support, and they are expected to do the basic and applied research that can be translated into new products and services by the private sector. But how many technologies are emerging from the science-based process of technology change?
Recent science-based innovations
There are many possible ways to measure the number of science-based technologies that have recently been successfully commercialized. Depending on the definition of “recently,” one could list post-World War II examples such as those mentioned above. However, in addition to the relatively old age of these examples, one would also like to have a relatively unbiased database to measure the recent emergence of science-based technologies. One way to produce such a database is to analyze patents obtained by successful start-ups to see how many of these patents cite scientific and engineering papers. Consider companies that are members of the Wall Street Journal‘s “Billion Dollar Startup Club,” most of which were founded between 2002 and 2012. They are global start-ups that have billion-dollar valuations, are still private, have raised money in the past four years, and have at least one venture-capital firm as an investor.
Table 1 shows the percentage of start-ups by the total numbers of science and engineering papers mentioned in their patents. Not only are well-known science-based technologies such as nanotechnology, quantum dots, superconductors, and quantum computers, or new forms of integrated circuits, displays, solar cells, and batteries, not represented in Table 1, only eight (6%) of the 143 start-ups cited more than 10 different scientific papers in their patents, and six of them (5%) are biotech and bio-electronic start-ups. The importance of advances in science to biotech start-ups is not surprising. Ninety percent of royalty income for the top 10 universities comes from biotechnology, and universities obtain a larger percentage of the patents awarded in biotech (about 9%) than for all other high-tech sectors (about 2%).
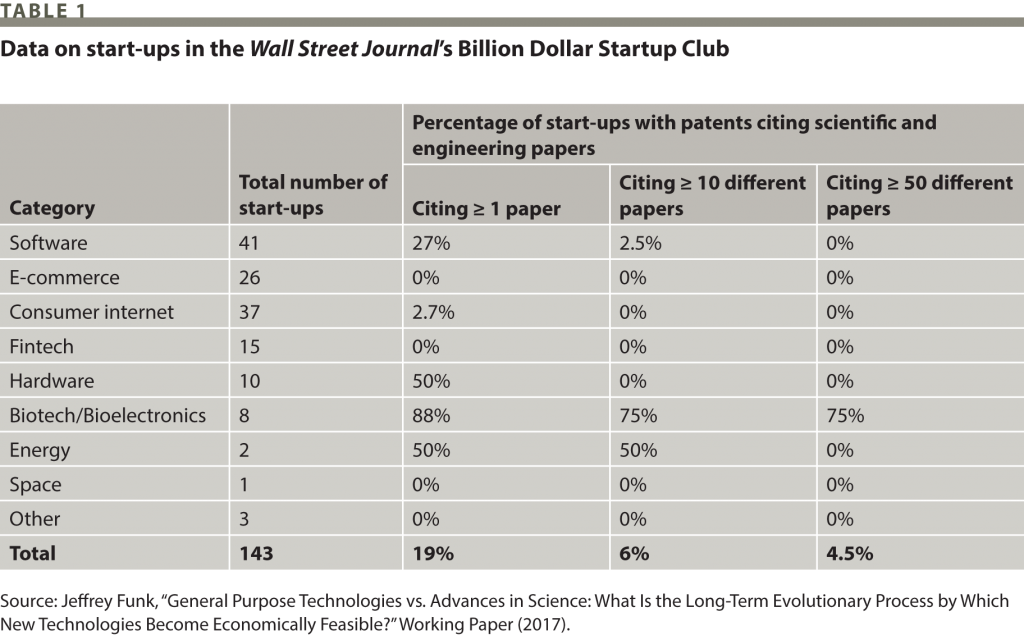
Some observers might argue that members of the billion-dollar start-up club probably licensed patents from other firms and thus are utilizing more ideas from scientific papers than are shown in Table 1. However, even doubling the number of papers cited in the patents would not significantly change the results, and these increases in paper numbers might not even equal the number of papers typically added by patent examiners. Even those papers cited by start-ups were mostly from engineering journals and not pure science journals such as Nature and Science. And when patents did cite previous information, they cited practitioner magazines, books, and blogs more than science and engineering papers, suggesting that most entrepreneurs are looking for information outside of science and engineering journals.
The small number of start-ups in the billion-dollar club that depend on advances in science or mention science and engineering papers in their patents is probably not surprising to most people in the private sector. It is well known that few academic papers are read and that patents are not relevant for most businesses. One study found that only 11% of e-commerce firms had applied for even a single patent as of 2012, as compared with 65% and 62%, respectively, for semiconductor and biotech firms. The percentage applying for patents is of course lower than the percentage receiving patents, and most of the e-commerce patent applications involve business models and few or none involve science-based patents. This provides further evidence that advances in science play a small role in successful start-ups such as the billion-dollar club members.
A second type of evidence supporting the case that few science-based technologies have recently been successfully commercialized comes from my analysis of predicted breakthrough technologies by MIT Technology Review between 2001 and 2005. Because these predictions reflect research activities, the market sizes provide insights into whether research done at leading universities in the 1990s and 2000s has become the basis for new products and services. As shown in Table 2, one predicted breakthrough (data mining) has greater than $100 billion in sales; three have between $10 billion and $50 billion (power grid control, biometrics, distributed storage); one has sales between $5 billion and $10 billion (micro-photonics); six have sales between $1 billion and $5 billion; eight have sales between $100 million and $1 billion; and 14 have sales of less than $100 million. (Data for seven could not be found.)
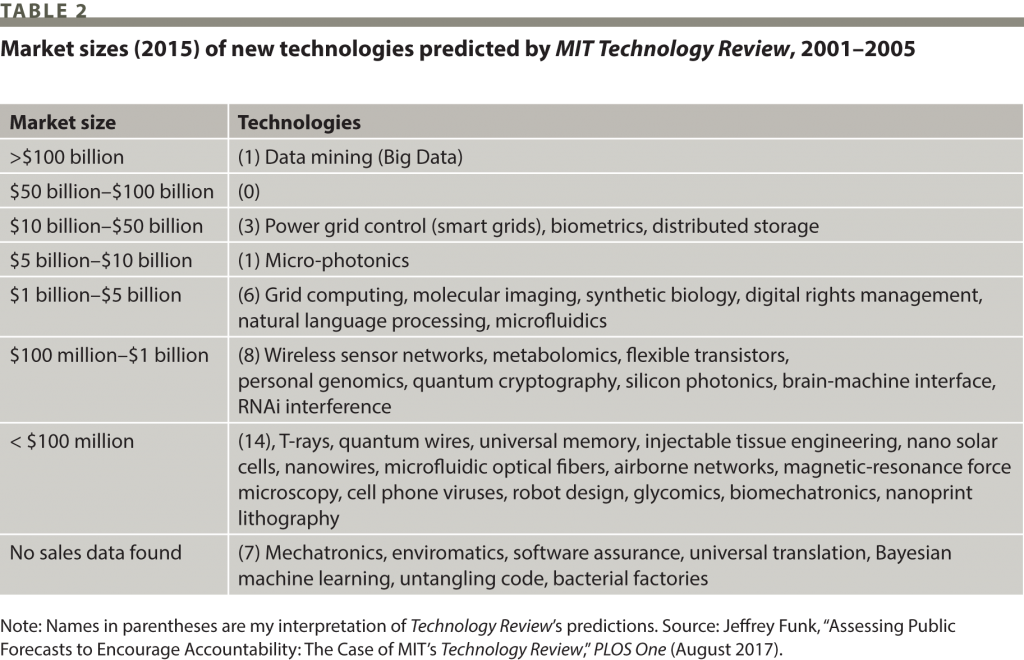
In comparison with other recently successful technologies that were not chosen by Technology Review (and for which there were no markets when the magazine made its predictions), the sizes of the markets for most of the predictions are very small. My own nonsystematic survey of a variety of high-tech industries—based on more than 20 years of working with hundreds of students and companies, as well as on reading Science, Nature, the Wall Street Journal, and websites on the year’s best new products, services, and technologies—identified three technologies with markets greater than $100 billion in 2015 (smartphones, cloud computing, Internet of Things), one with between $50 billion and $100 billion (tablet computers), and four (social networking, fintech, eBooks, wearable computing) with between $10 billion and $50 billion (see Table 3). In other words, eight technologies had greater than $10 billion in sales as compared with only four of Technology Review‘s predictions. Thus, the predictions do not capture a lot of what makes it big in the marketplace, and most of the predicted technologies do not end up having much economic importance.
The second and related interesting thing about the magazine’s predictions is that most of them are science-based technologies, a finding that should not be surprising since high-end research universities such as the Massachusetts Institute of Technology (MIT), which publishes Technology Review, build their reputations in part on claims that basic science performed by their faculty make critical contributions to technological advance. The numbers of papers published in top science and engineering journals and the number of times these papers are cited are standard measures of support for such claims. It is only natural that MIT and other high-end universities would emphasize science-based technologies when they predict breakthrough technologies and when they look for opportunities in general.
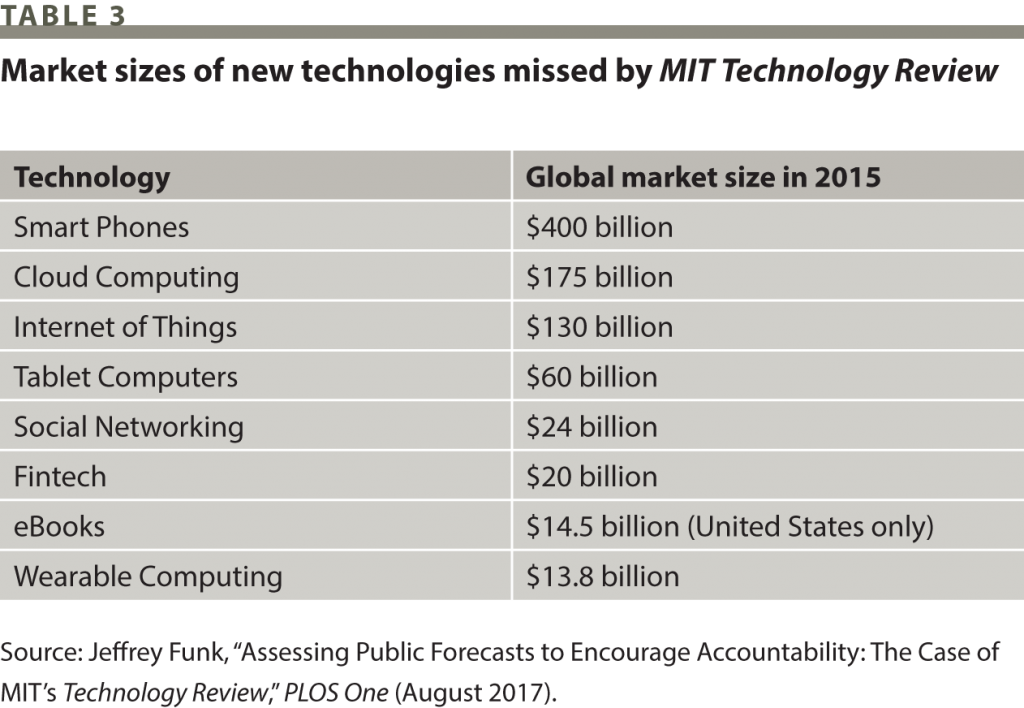
Support for this interpretation comes from the names of the breakthrough technologies chosen by Technology Review. Many of them sound more like research disciplines than products or services: metabolomics, T-rays (terahertz rays), RNAi interference, glycomics, synthetic biology, quantum wires, quantum cryptography, robot design, and universal memory. Contrast the names of these predicted breakthrough technologies with successful ones missed by the magazine (such as smartphones, cloud computing, Internet of Things, tablet computers, social networking, fintech, and eBooks) and the differences between science-based and Silicon Valley innovation become more apparent. The emergence and evolution of research disciplines represent an early step in the science-based process of technology change, one that often occurs in the university setting, but this step cannot easily be extrapolated to marketplace impact, as shown by the modest performance of most of the Technology Review predictions.
Furthermore, a focus on the science-based process of technology change may also be a major reason why market data were not found for seven of the predictions: mechatronics, enviromatics, software assurance, universal translation, Bayesian machine learning, untangling code, and bacterial factories. Most of these terms refer to broad sets of techniques that existed long before the magazine made its predictions, and thus they are more consistent with research disciplines than technologies that might form the basis for new products and services.
The Silicon Valley process of technology change
The Silicon Valley process of technology change represents a different process by which technologies become economically feasible. Rapid improvements in integrated circuits, lasers, photo-sensors, computers, the Internet, and smartphones enabled new forms of products, services, and systems to emerge in which Moore’s Law is the most well-known contributor to these rapid improvements. Some economists call these technologies “general purpose” technologies because they have a large impact on many economic sectors.
For example, consider the iPhone, whose design and architecture did not directly depend on advances in basic or applied science or on publications in academic journals; it can also be defined as a general purpose technology. My 2017 paper in Industrial & Corporate Change quantitatively examines how improvements in microprocessors, flash memory, and displays enabled the iPhone, Apple’s app store, and specific types of apps to become economically feasible. Certain performance and price points for flash memory were necessary before iPhones could store enough apps (along with pictures, videos, and music) to make Apple’s app store feasible. Certain levels of microprocessor price and performance were needed before the iPhone could quickly and inexpensively process 3G signals when apps or content were downloaded. Even iPhone touch screen displays represent recent improvements (a new touch-screen layer) in an overall trajectory for LCD displays. By enabling the iPhone and app store to become economically feasible, these improvements also enabled a broad number of app services to become feasible, such as ride sharing, room booking (Airbnb), food delivery, mobile messaging (WhatsApp), and music services.
The impact of rapid improvements in Internet speed and cost on the emergence of new products and services has also been large. For example, these improvements changed the economics of placing images, videos, and objects (for example, Flash files) on web pages. In the late 1990s, web pages could not include them because downloading them was too expensive and time-consuming for users. But as improvements in Internet speed and cost occurred, for both wireline and wireless services, most websites added images, video, and objects, thus enabling aesthetically pleasing pages and the sale of items (such as fashion and furniture) that require high-quality graphics. Rapid improvements in Internet speed have also enabled cloud computing, Big Data services, and new forms of software including more complex forms of advertising, pricing, and recommendation techniques.
These types of rapid improvements and their resulting economic impact on new products, services, and systems represent a different process of technology change than does the science-based process. The Silicon Valley process of technology change primarily involves private firms, the resulting new products and services do not directly depend on advances in basic or applied science, and the ideas for them are not published in science and engineering journals.
Recent innovations from Silicon Valley
The Silicon Valley process of technology change has been critically important for the US economy. Improvements in microprocessors and memory chips (which benefited from Moore’s Law) led to technologies that include mini-computers in the 1960s; digital watches, calculators, and PCs in the 1970s; laptops, cellular phones, and game consoles in the 1980s; set-top boxes, web browsers, digital cameras, and PDAs in the 1990s; and MP3 players, e-book readers, digital TVs, smartphones, and tablet computers in the 2000s. Many new types of content and services have also emerged from improvements in Internet speed in the 1990s and 2000s, including music streaming and downloads, video streaming and downloads, cloud computing, software-as-a service, online games, social networking, and online education.
To analyze more recent examples, consider again the Wall Street Journal‘s billion-dollar start-up club. As discussed above, few of the start-ups’ patents cited scientific and engineering papers, and most of the cited papers were in engineering and not pure science journals. Evidence that many of the opportunities emerged from the Silicon Valley process of technology change can be seen in the large number of Internet-related start-ups in Table 1. Of the 143 start-ups, 119 offer Internet-related services; this roster includes 41 software, 26 e-commerce, 37 consumer Internet, and 15 fintech start-ups. Although advances in science are still important for parts of the Internet, such as photonics and lasers, by the 1990s the overall design of the Internet did not depend on these advances, and the emergence of new services depended mostly on improvements in a combination of Internet speed and cost and on new access devices such as smartphones. For example, certain levels of Internet speed and cost were necessary before cloud computing and Big Data services became economically feasible, and these two technologies form the basis for most of the software and fintech start-ups in Table 1. Also, most of the e-commerce start-ups in Table 1 (such as apparel sites) couldn’t successfully sell their products over the Internet until inexpensive and fast Internet services enabled the use of aesthetically pleasing and high-resolution images, videos, and flash content beginning in the mid-2000s. And many of the consumer Internet start-ups are apps that became feasible as inexpensive iPhones and Android phones became widely available.
Of course, the large number of Internet-related start-ups exploited by the billion-dollar club members should not be surprising since the Internet has long been a target for start-ups, peaking in 2001 during the so-called Internet bubble, just when Technology Review began predicting breakthrough technologies. Since 2001, according to various venture capital analyses, such as Dow Jones Venture Source 2016 and Wilmer-Hale’s 2016 Venture Capital Report, the fraction of total start-ups represented by life science (including bio-pharmaceutical, medical devices, and Internet-related ones) has increased slightly both in terms of financing announcements and financing dollars. Nevertheless, even in 2015, only 22% of start-ups were classified as health care-related financings, while 71% were Internet and electronic hardware financings. The 71% figure is slightly less than the 83% figure (119/143) for the billion-dollar club, suggesting that the chances of success for Internet firms may be slightly higher than for non-Internet firms.
These data suggest that many more products and services, and much more economic activity, have recently emerged successfully from the Silicon Valley than from the science-based process of technology change. Most of the innovation in the US economy seems to be concentrated in those sectors for which information technology has a large impact, such as computing, communications, entertainment, finance, and logistics, with transportation (ride sharing and driverless vehicles) perhaps joining this group in the near future.
This conclusion is consistent with the pessimistic assessments of the US economy offered by the economists Robert Gordon and Tyler Cowen. Both argue that recent technological change has been concentrated in a few sectors, and both question whether information technology, including Big Data and artificial intelligence, can have a positive impact on a wider group of sectors in the future. Their views reinforce a widely held belief—which I share—that productivity improvements in other sectors require science-based innovations, which in turn means that the United States’ economic future depends on improved processes of science-based technology change. Advances in genetically modified organisms and synthetic food are needed for the food sector; advances in nanomaterials are needed for the housing, automobiles, aircraft, electronics, and other sectors; advances in new types of solar cells (such as quantum dots and Perovskites), fuel cells, batteries, and superconductors are needed for the energy sector; advances in electronics and computing are needed to revive Moore’s Law; and advances in science’s understanding of human biology are needed to extend healthful longevity.
This means that improving the science-based process of technology change is a critical task for the United States and its allies. Not only have relatively few science-based technologies recently been successfully commercialized, but this performance record stands in contrast with the dramatic increases in recent decades in funding for basic research, particularly at universities, that are often justified in terms of their potential to catalyze technological advance. Expenditures from the US government on “basic research” rose from $265 million in 1953 to $38 billion in 2012, or 20 times higher when adjusted for inflation. For basic research at universities and colleges, the expenditures rose by more than 40 times over this period, from $82 million to $24 billion. Dramatically higher expenditures and lower output suggest that this process is not working as well as it should. This conclusion is consistent with other research showing significant declines in the productivity of biomedical innovation—a phenomenon described by one analyst as Eroome’s (that’s Moore’s spelled backward) Law. Furthermore, the greater success of the Silicon Valley process of technology change suggests that the problems with the science-based process lie more in the upstream (university) than in the downstream (private sector) side.
Making science valuable
The previous sections illustrate several problems with the science-based process of technology change. First, most of the output from university research is academic papers, yet few corporate engineers and scientists cite science and engineering papers in patents, or probably even read these papers. The well-known business magnate, engineer, and inventor Elon Musk has publicly said that most academic papers are useless. Others argue that government, environment, and health care professionals also do not read these papers or use them to make policy. Asit Biswas, a member of the World Commission on Water for the 21st Century—who has also been a senior adviser to 19 governments and six heads of United Nations agencies, and brandishes 8,773 citations, an h-index of 39, and meetings with three popes—observed in a recent op-ed: “We know of no senior policymaker or senior business leader who ever read regularly any peer-reviewed papers in well-recognized journals like Nature, Science or Lancet.”
There are probably many reasons why few academic papers are read and used by policy makers and private-sector managers, engineers, and scientists. The biggest reason is probably that their value does not exceed the problems of accessibility and cost, which are quite high for most people. The value is low because criteria used by academic reviewers and private-sector engineers and scientists to evaluate new technologies are very different. Academic reviewers emphasize scientific explanations, elegant mathematics, comprehensive statistics, and full literature reviews while private-sector engineers and scientists emphasize performance and cost.
A second reason is that many pre-commercialization improvements are needed before these technologies can be commercialized, as I showed in my 2015 Research Policy analysis. Although management books prattle on about the shorter time needed to commercialize new technologies than in the past, the low output of science-based technologies along with the productivity slowdown documented by Robert Gordon and Tyler Cowen suggest otherwise. The reality is that these technologies take many decades if not longer to be commercialized, during which time many improvements must be implemented. For example, the phenomena of photovoltaics and electro-luminescence were discovered in the 1840s and early 1900s, respectively, yet the diffusion of solar cells and LEDs has been quite recent even as they experienced very rapid improvements over the past 50 years. If the United States is to increase the rate of commercialization for science-based technologies, these time periods must be reduced through new ways of doing government-sponsored research. How will this be achieved?
One way is to create stronger links between advances in science and the achievement of particular technical or social goals. Such a mission-based approach to research and development (R&D) was central to how, during the Cold War, the US Department of Defense successfully developed fighter aircraft, bombers, jet engines, the atomic bomb, transistors, integrated circuits, magnetic disks, computers, GPS, and the Internet. The department emphasized improvements in cost and specific dimensions of performance in which the quest to improve technological performance often required researchers to devise new scientific explanations. The department still uses this approach in its Defense Advanced Research Projects Agency, which can count drones and driverless vehicles as two recent successes.
A mission-oriented approach to scientific research can be applied to a wide variety of technologies with applications in areas such as health and the environment. In a mission-based approach, decision makers fund technology that can enable measurable improvements in various products and services along with health or environmental outcomes. Crucially, such mission-based R&D must fund multiple approaches and multiple recipients for each technology, while emphasizing reproducible improvements more than academic publications, over time scales that are longer than those typically demanded in the marketplace, but shorter than those typically experienced for science-based technological change. Recipients that provide measurable improvements should be rewarded with more funding, while those that do not are not rewarded.
If a future of slower growth and more inequity predicted by thinkers such as Gordon and Cowan is to be avoided, US policy makers should be moving more of the nation’s R&D investment toward a mission-based approach, and they should also be experimenting with different approaches to implementation. Three leading economists of innovation, David Mowery, Richard Nelson, and Ben Martin, argued recently in a Research Policy paper that an important issue in an R&D system is the “balance between decentralization and centralization in program structure and governance.” A mission-based approach represents more centralization than does the current decentralized system of academics writing papers, but what level of centralization is most appropriate, and how should it be administered? How can scientific creativity be maintained and promoted while also linking it to problem solving? How should technological choices be made, and how should different institutions in academia, government, and the private sector work together in making such choices? If public R&D funds are not going to increase significantly in the next several years, as seems to be the case, how should the proper balance between mission-based approach and the existing decentralized network of professors writing papers be established? Science-based technology’s contributions to economic growth and social problem solving are inadequate. Policy makers need to begin experimenting with new ways to improve its performance.