The Limits of Data
Data is powerful because it’s universal. The cost is context.
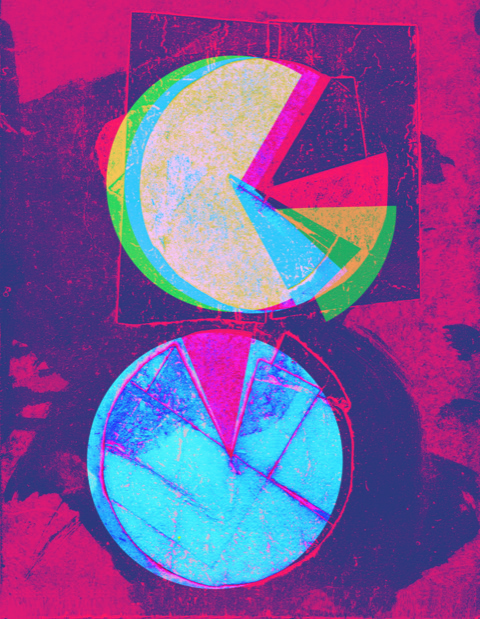
I once sat in a room with a bunch of machine learning folks who were developing creative artificial intelligence to make “good art.” I asked one researcher about the training data. How did they choose to operationalize “good art”? Their reply: they used Netflix data about engagement hours.
The problem is that engagement hours are not the same as good art. There are so many ways that art can be important for us. It can move us, it can teach us, it can shake us to the core. But those qualities aren’t necessarily measured by engagement hours. If we’re optimizing our creative tools for engagement hours, we might be optimizing more for addictiveness than anything else. I said all this. They responded: show me a large dataset with a better operationalization of “good art,” we’ll use it. And this is the core problem, because it’s very unlikely that there will ever be any such dataset.
Right now, the language of policymaking is data. (I’m talking about “data” here as a concept, not as particular measurements.) Government agencies, corporations, and other policymakers all want to make decisions based on clear data about positive outcomes. They want to succeed on the metrics—to succeed in clear, objective, and publicly comprehensible terms. But metrics and data are incomplete by their basic nature. Every data collection method is constrained and every dataset is filtered.
Some very important things don’t make their way into the data. It’s easier to justify health care decisions in terms of measurable outcomes: increased average longevity or increased numbers of lives saved in emergency room visits, for example. But there are so many important factors that are far harder to measure: happiness, community, tradition, beauty, comfort, and all the oddities that go into “quality of life.”
Consider, for example, a policy proposal that doctors should urge patients to sharply lower their saturated fat intake. This should lead to better health outcomes, at least for those that are easier to measure: heart attack numbers and average longevity. But the focus on easy-to-measure outcomes often diminishes the salience of other downstream consequences: the loss of culinary traditions, disconnection from a culinary heritage, and a reduction in daily culinary joy. It’s easy to dismiss such things as “intangibles.” But actually, what’s more tangible than a good cheese, or a cheerful fondue party with friends?
It’s tempting to use the term intangible when what we really mean is that such things are hard to quantify in our modern institutional environment with the kinds of measuring tools that are used by modern bureaucratic systems. The gap between reality and what’s easy to measure shows up everywhere. Consider cost-benefit analysis, which is supposed to be an objective—and therefore unimpeachable—procedure for making decisions by tallying up expected financial costs and expected financial benefits. But the process is deeply constrained by the kinds of cost information that are easy to gather. It’s relatively straightforward to provide data to support claims about how a certain new overpass might help traffic move efficiently, get people to work faster, and attract more businesses to a downtown. It’s harder to produce data in support of claims about how the overpass might reduce the beauty of a city, or how the noise might affect citizens’ well-being, or how a wall that divides neighborhoods could erode community. From a policy perspective, anything hard to measure can start to fade from sight.
An optimist might hope to get around these problems with better data and metrics. What I want to show here is that these limitations on data are no accident. The basic methodology of data—as collected by real-world institutions obeying real-world forces of economy and scale—systematically leaves out certain kinds of information. Big datasets are not neutral and they are not all-encompassing. There are profound limitations on what large datasets can capture.
The basic methodology of data—as collected by real-world institutions obeying real-world forces of economy and scale—systematically leaves out certain kinds of information.
I’m not just talking about contingencies of social biases. Obviously, datasets are bad when the collection procedures are biased by oversampling by race, gender, or wealth. But even if analysts can correct for those sorts of biases, there are other, intrinsic biases built into the methodology of data. Data collection techniques must be repeatable across vast scales. They require standardized categories. Repeatability and standardization make data-based methods powerful, but that power has a price. It limits the kinds of information we can collect.
A small group of scholars have been working on understanding this, mostly in science and technology studies—an interdisciplinary field focused on how science works that conducts studies across philosophy, history, anthropology, sociology, and more. This work offers an understanding of the intrinsic limitations on the process of data collection and on the contents of big datasets. And these limitations aren’t accidents or bad policies. They are built into the core of what data is. Data is supposed to be consistent and stable across contexts. The methodology of data requires leaving out some of our more sensitive and dynamic ways of understanding the world in order to achieve that stability.
These limitations are particularly worrisome when we’re thinking about success—about targets, goals, and outcomes. When actions must be justified in the language of data, then the limitations inherent in data collection become limitations on human values. And I’m not worried just about perverse incentives and situations in which bad actors game the metrics. I’m worried that an overemphasis on data may mislead even the most well-intentioned of policymakers, who don’t realize that the demand to be “objective”—in this very specific and institutional sense—leads them to systematically ignore a crucial chunk of the world.
Decontextualization
Not all kinds of knowledge, and not all kinds of understanding, can count as information and as data. Historian of quantification Theodore Porter describes “information” as a kind of “communication with people who are unknown to one another, and who thus have no personal basis for shared understanding.” In other words, “information” has been prepared to be understood by distant strangers. The clearest example of this kind of information is quantitative data. Data has been designed to be collected at scale and aggregated. Data must be something that can be collected by and exchanged between different people in all kinds of contexts, with all kinds of backgrounds. Data is portable, which is exactly what makes it powerful. But that portability has a hidden price: to transform our understanding and observations into data, we must perform an act of decontextualization.
Data must be something that can be collected by and exchanged between different people in all kinds of contexts, with all kinds of backgrounds.
An easy example is grading. I’m a philosophy professor. I issue two evaluations for every student essay: one is a long, detailed qualitative evaluation (paragraphs of written comments) and the other is a letter grade (a quantitative evaluation). The quantitative evaluation can travel easily between institutions. Different people can input into the same system, so it can easily generate aggregates and averages—the student’s grade point average, for instance. But think about everything that’s stripped out of the evaluation to enable this portable, aggregable kernel.
Qualitative evaluations can be flexible and responsive and draw on shared history. I can tailor my written assessment to the student’s goals. If a paper is trying to be original, I can comment on its originality. If a paper is trying to precisely explain a bit of Aristotle, I can assess it for its argumentative rigor. If one student wants be a journalist, I can focus on their writing quality. If a nursing student cares about the real-world applications of ethical theories, I can respond in kind. Most importantly, I can rely on our shared context. I can say things that might be unclear to an outside observer because the student and I have been in a classroom together, because we’ve talked for hours and hours about philosophy and critical thinking and writing, because I have a sense for what a particular student wants and needs. I can provide more subtle, complex, multidimensional responses. But, unlike a letter grade, such written evaluations travel poorly to distant administrators, deans, and hiring departments.
Quantification, as used in real-world institutions, works by removing contextually sensitive information. The process of quantification is designed to produce highly portable information, like a letter grade. Letter grades can be understood by everybody; they travel easily. A letter grade is a simple ranking on a one-dimensional spectrum. Once an institution has created this stable, context-invariant kernel, it can easily aggregate this kind of information—for students, for student cohorts, for whole universities. A pile of qualitative information, in the form of thousands of written comments, for example, does not aggregate. It is unwieldy, bordering on unusable, to the administrator, the law school admissions officer, or future employer—unless it has been transformed and decontextualized.
So here is the first principle of data: collecting data involves a trade-off. We gain portability and aggregability at the price of context-sensitivity and nuance. What’s missing from data? Data is designed to be usable and comprehensible by very different people from very different contexts and backgrounds. So data collection procedures tend to filter out highly context-based understanding. Much here depends on who’s permitted to input the data and who the data is intended for. Data made by and for specialists in forensic medicine, let’s say, can rely on a shared technical background, if not specific details of working in a particular place or a particular community.
The clearest cases of decontextualization are with public transparency, where a data-based metric needs to be comprehensible to all. Sociologist Jennifer Lena provides an excellent example from the history of arts funding. Assessing which art projects are worthwhile and deserve funding depends on an enormous amount of domain-specific expertise. To tell what’s original, creative, and striking requires knowing a lot about the specific medium and genre in question, be it film, comics, or avant-garde performance art. And there’s not really such a thing as generic expertise in art criticism. Being a jazz expert gives you no insight into what’s exciting in the world of indie video games.
But transparency metrics tend to avoid relying on specialized domain expertise, precisely because that expertise isn’t accessible to the public at large. Lena writes that when Congress became worried about the possibility of nepotism and corruption in the National Endowment for the Arts’ funding decisions, it imposed an accountability regime that filtered out expert knowledge in exchange for a simple, publicly comprehensible metric: ticket sales. The problem should be obvious: blockbuster status is no measure of good art. But ticket sales are easy to measure, easy to aggregate, and easy to comprehend on the largest of scales.
The wider the user base for the data, the more decontextualized the data needs to be. Theodore Porter’s landmark book, Trust in Numbers, gives a lovely example drawn from a history of land measurement compiled by Witold Kula, the early twentieth-century Polish economist. Older measures of land often were keyed to their productivity. For example, a “hide” of land was the amount required to sustain the average family. Such measures are incredibly rich in functional information. But they required a lot of on-the-ground, highly contextual expertise. The land assessor needs to understand the fertility of the soil, how many fish are in the rivers and deer are in the woods, and how much all that might change in a drought year. These measures are not usable and assessable by distant bureaucrats and managers. Societies tend to abandon such measures and switch from hides to acres when they shift from local distributed governance to large, centralized bureaucracies. The demands of data—and certainly data at scale—are in tension with the opacity of highly local expertise and sensitivity. This kind of local awareness is typically replaced with mechanically repeatable measures in the movement to larger-scaled bureaucracy.
The wider the user base for the data, the more decontextualized the data needs to be.
Behind such shifts is the pressure to be objective in a very particular way. There are many different meanings for “objective.” Sometimes when we say something is “objective,” we mean that it’s accurate or unbiased. But other times, we’re asking for a very specific social transformation of our processes to fit our institutional life. We are asking for mechanical objectivity—that is, that a procedure be repeatable by anybody (or anybody with a given professional training), with about the same results. Institutional quantification is designed to support procedures that can be executed by fungible employees.
This mechanical objectivity has become central to contemporary institutional life. It’s easy to forget that mechanical objectivity isn’t everything. People often assume, for instance, that if you have mechanical objectivity, then you have accuracy—but these are different things. An accurate judgment gets at what really matters. But the methodology that leads to the most accurate judgments may not scale. Consider, for example, the legal standard for charging somebody with driving under the influence when the person has a blood alcohol level of 0.08%. This isn’t the most reliable guide to assessing what really matters, which is inebriation to the point of impairment. As it turns out, some people are impaired at lower blood alcohol levels, and some are impaired at higher ones. But it’s very hard to find a scalable and repeatable procedure to judge impairment. So we use the 0.08% blood-alcohol standard because anybody with a breathalyzer can apply it with approximately the same results.
Consider, too, the relationship between the complex idea of “adulthood” and the more mechanical idea of “legal age.” The right to vote, the ability to give consent, and all the other associated rights of adulthood should probably be keyed to intellectual and emotional maturity. But there’s no mechanically objective way to assess that. Some particular people might be good at assessing intellectual and emotional maturity, especially in those they know well. But those procedures don’t scale. So countries like the United States peg the right to vote to a very simple standard—18 years of age—in order to achieve mechanical objectivity.
The historian Lorraine Daston puts it this way: older forms of rules often permitted enormous amounts of discretion and judgment. But in the last few centuries, complex judgment has been replaced with clear and explicit rules—what she calls “algorithmic rules.” Algorithmitization wasn’t initially intended to make information machine-calculable, but instead to cheapen labor, to replace highly trained specialists with low-skilled and replaceable workers who could simply execute an explicit set of rules. The problem, argues Daston, is that explicit and mechanical rule sets only do well when contexts don’t change very much.
The first lesson, again, is that data involves a trade-off. The power of data is that it is collectible by many people and formatted to travel and aggregate. The process of making data portable also screens off sensitive, local, or highly contextual modes of understanding. In transforming understanding into data, we typically eliminate or reduce evaluative methods that require significant experience or discretionary judgment in favor of methods that are highly repeatable and mechanical. And if policymakers insist on grounding their policy in large-scale public datasets, then they are systematically filtering out discretion, sensitivity, and contextual experience from their decisionmaking process.
The politics of classification
Data collection efforts require classification, which is a second kind of filter. Imagine a US census form where everybody simply wrote into a blank space their racial identity, in their own terms. There would be no way to aggregate this easily. Collectors need to sort information into preprepared buckets to enable aggregation. So there are distinct buckets—white, Black, American Indian, Asian, and, in the recent census, “Two or More”—which organize a complex spectrum into a discrete set of chunks. We either presort people’s responses into those buckets by forcing them to choose from a limited list, or we sort them into categories after the fact by coding their free responses.
Informatics scholar Geoffrey Bowker and science studies scholar Susan Leigh Star offer a profound analysis of these pressures in Sorting Things Out: Classification and its Consequences, their political history of classification systems. The buckets that data collectors set up constitute a kind of intentional, institutional forgetting. Sorting information into categories emphasizes information at the boundaries—say, the difference between white and Asian—and puts that information into storage. But those categories also act as a filter; they don’t store information inside the buckets. The US Census categories, for example, elide the difference between Korean, Chinese, Filipino, Khmer, and more—they’re all lumped into “Asian.” This lumping is of necessity, say Bowker and Star: the process of classification is designed to wrangle the overwhelming complexity of the world into something more manageable—something tractable to individuals and institutions with limited storage and attentional capacity. Classification systems decide, ahead of time, what to remember and what to forget.
All classification systems are the result of political and social processes, which involve decisions about what’s worth remembering and what we can afford to forget.
But these categories aren’t neutral. All classification systems are the result of political and social processes, which involve decisions about what’s worth remembering and what we can afford to forget. Some of these constraints are simply practical. Early mortality data collection, write Bowker and Star, was limited by the maximum size of certain forms: you couldn’t have more causes of death than there were lines in the standard form. And it’s very hard to add new causes of death to the data collection system because such an effort would involve convincing hundreds of different national data collection offices to change all their separate death reporting forms.
Here is the second principle: every classification system represents some group’s interests. Those interests are often revealed by where a classification system has fine resolution and where it doesn’t. For example, the International Classification of Disease (ICD) is a worldwide, standardized system for classifying diseases that’s used in collecting mortality statistics, among other things. Without a centralized, standardized system, the data collected by various offices won’t aggregate. But the ICD has highly variable granularity. It has separate categories for accidents involving falling from playground equipment, falling from a chair, falling from a wheelchair, falling from a bed, and falling from a commode. But it only has two categories for falls in the natural world: fall from a cliff, and an “other fall” category that lumps together all the other falls—including, in its example, falls from embankments, haystacks, and trees. The ICD is obviously much more interested in recording the kinds of accidents that might befall people in an urban industrial environment than a rural environment, note Bowker and Star. The ICD’s classification system serves some people’s interests over others.
Classification systems decide ahead of time what to remember and what to forget. This is not bad in and of itself, argue Bowker and Star. Data aggregation requires such filtering. The problem occurs when users of data forget that categories are social inventions created for a purpose. When these classificatory schemes enter an information infrastructure, they become invisible; they become part of the background operating structure of our world. People start assuming that Asian and white and Black are natural categories, and those assumptions quietly reshape the world we live in.
Political interests shape classifications systems, and classification systems shape every institutional data-gathering effort. The government collects data on where citizens live, how much they earn, what property they own. Grocery store chains collect information on what consumers purchase and when. Medical insurance companies collect information on the insured person’s heart rate, temperature, and official medical diagnosis every time the person has an official interaction with medical institutions. Each of these institutions uses an information infrastructure, which is set up to record some very specific kinds of information—but which also makes it difficult to record anything else.
Sometimes information infrastructures do offer a place for unstructured notes. When I’m entering my grades into the school’s database, I get a little blank box for other notes. The information is collected in some sense, but it doesn’t really move well; it doesn’t aggregate. The system aggregates along the classificatory lines that it has been prepared to aggregate. I may offer the system important contextual information, but the aggregating system will usually filter that stuff out; there’s not much sign of it by the time the high-level decisionmakers get their benchmarks and metrics. Unstructured information isn’t legible to the institution. We who enter information into data systems can often feel their limitations, so we try to add richness and texture—which the system nominally collects and then functionally ignores.
Data collection efforts aren’t neutral and they aren’t complete. They emphasize a particular style of knowledge formatted in a particular way, which makes it possible for the data to slide effortlessly between contexts, be gathered by all sorts of different people for use across vast scales. There is a cost to be paid for this scalability, this independence from context. The data collection methodology tends to filter out the personal, the intimate, the special understanding.
Metrics and values
The consequences of that cleansing are perhaps clearest in the cases of metrics and other data-driven targets. Consider transparency metrics. I’ve argued that transparency schemes have a clear price; transparency is a kind of surveillance. Public transparency requires that the reasoning and actions of institutional actors be evaluated by the public, using metrics comprehensible to the public. But this binds expert reasoning to what the public can understand, thus undermining their expertise. This is particularly problematic in cases where the evaluation of success depends on some specialized understanding. The demand for public transparency tends to wash deep expertise out of the system. Systems of transparency tend to avoid evaluative methods that demand expertise and sensitivity and instead prefer simple, publicly comprehensible standards—such as ticket sales or graduation rates or clicks.
Systems of transparency tend to avoid evaluative methods that demand expertise and sensitivity and instead prefer simple, publicly comprehensible standards.
This isn’t to say that transparency is bad. The demand for data-based transparency is an incredibly powerful and effective tool for fighting bias and corruption. But this demand also exposes us to a set of costs. Transparency metrics are based on publicly comprehensible data. Consider the case of Charity Navigator, which promises to guide your donation dollars by ranking the effectiveness of various nonprofits. For years, Charity Navigator’s rankings were heavily based on an “overhead ratio”—a measure of how much donated money made it through to an external goal compared to how much was spent internally, as overhead. This seems like a great measure of efficiency, and Charity Navigator became a dominant force in guiding donations to nonprofits. But as many experts from the nonprofit realm have complained, the overhead ratio measure is flawed and misleading. Suppose a nonprofit promises to help improve water purification in impoverished areas. Distributing water purification machinery counts as an external expenditure, so it improves the organization’s overhead ratio. But hiring an expert in waterborne bacteria or building a better internal database for tracking long-term use of that purification machinery counts as an internal cost—and so drops the organization’s ranking.
Understanding what’s important generally takes spending an enormous amount of expertise and time within that particular domain. The late anthropologist Sally Engle Merry explored a particularly devastating example in her 2016 book, The Seductions of Quantification. At the time, she reported, international attempts to reduce sex trafficking revolved around tracking success with a single clear metric, generated by the US State Department, in the Trafficking in Persons (TIPS) report. That measure, Merry related, was based on the conviction rate of sex traffickers. This may make sense to the uninitiated, but to experts in the subject, it’s a terrible metric. Sex trafficking is highly related to ambient poverty. If a country reduced ambient poverty, doing so typically reduces sex trafficking. But this would show up in the TIPS report as a failure to control sex trafficking. If sex trafficking dropped due to economic reasons, there would be fewer sex traffickers to convict. The TIPS report had come to dominate the international conversation, Merry wrote, because actual sex trafficking is extremely hard to measure while conviction rates are quite easy to collect.
This dangerous separation of metric from meaning accelerates when people internalize certain metrics as core values. I have called this process “value capture”: when our deepest values get captured by institutional metrics and then become diluted or twisted as a result. Academics aim at citation rates instead of real understanding; journalists aim for numbers of clicks instead of newsworthiness. In value capture, we outsource our values to large-scale institutions. Then all these impersonal, decontextualizing, de-expertizing filters get imported into our core values. And once we internalize those impersonalized values as our own, we won’t even notice what we’re overlooking.
And now, in the algorithmic era, there’s a new version of this problem: these filtered values will be built so deeply into the infrastructure of our technological environment that we will forget that they were filtered in the first place. As artificial intelligence ethicists Sina Fazelpour and David Danks put it, target-setting is one of the most important—but most neglected—entry points for algorithmic bias. Let’s say programmers are training a machine learning model to help improve some quality: reduce crime, for instance, or make good art. Many contemporary training procedures involve randomly generating variations on a model and then pitting them against each other to see which one better hits the target. Fazelpour and Danks discuss a real-world case in which machine learning algorithms were trained to predict student success. But the training procedure itself can introduce biases, depending on who sets the targets, and which targets they select. In this case, the training targets were set by administrators and not students, thereby reflecting administrator interests. “Student success” was typically operationalized in terms of things like graduation rate and drop-out rate, rather than, say, mental health or rich social experiences. The machine learning algorithms were trained to hit a target—but the target itself can be biased.
In the algorithmic era, there’s a new version of this problem: these filtered values will be built so deeply into the infrastructure of our technological environment that we will forget that they were filtered in the first place.
Lessons from Porter apply here as well. To train a machine learning algorithm, engineers need a vast training dataset in which successes and failures are a clear part of the dataset. It’s easy to train a machine learning algorithm to predict which students will likely get a high grade point average, graduate quickly, or get a job afterwards. It’s easy to have a mechanical, repeatable, scalable procedure to accurately evaluate graduation speed, and very hard to have a mechanical, repeatable, and scalable procedure to accurately evaluate increased thoughtfulness. Nor are there large datasets that can train a machine learning algorithm to predict which students will become happier, wiser, or more curious as a result of their education. What algorithms can target depends on what’s in the datasets—and those datasets are tuned to what can be easily, mechanically collected at scale.
The more opaque the training procedure for algorithms, the more hidden these specific, biased, and political decisions will be in setting the targets. The more distant the users are from the training process, the easier it will be for them to assume that the algorithm’s outputs are straightforwardly tracking the real thing—student success—and the easier it will be to forget that the demands of institutional data collection have already filtered out whole swathes of human life and human value.
What can we do?
My point isn’t that we should stop using data-based methods entirely. The key features of data-based methodologies—decontextualization, standardization, and impersonality—are precisely what permit the aggregation of vast datasets and are crucial to reap the many rewards of data-based methodologies.
But policymakers and other data users need to keep in mind the limitations baked into the very essence of this powerful tool. Data-based methods are intrinsically biased toward low-context forms of information. And every data collection method requires a system of standardization that represents somebody’sinterest.
This suggests at least two responses to the limitations of data. First, when confronted with any large dataset, the user should ask: Who collected it? Who created the system of categories into which the data is sorted? What information does that system emphasize, and what does it leave out? Whose interests are served by that filtration system?
Who created the system of categories into which the data is sorted? What information does that system emphasize, and what does it leave out? Whose interests are served by that filtration system?
These are ordinary questions. In ordinary social situations, we know enough to ask basic questions: What are the motivations of a speaker? What are his interests and what are his biases? These same basic suspicions should also be applied to data. It’s tempting, however, to see datasets as somehow magically neutral and free of informational gaps. Maybe this is because when a person is talking to us, it’s obvious that there’s a personality involved—an independent agent with her own interests and motivations and schemes. But data is often presented as if it arose from some kind of immaculate conception of pure knowledge.
As Merry puts it, metrics and indicators require all kinds of political compromises and judgment calls to compress so much rich information into a single ranking. But the superficially simple nature of the final product—the metric—tends to conceal all kinds of subjectivity and politics. This is why, as Porter observes, public officials and bureaucrats often prefer justification in terms of such metrics. The numbers appear fair and impartial. Writes Porter: “Quantification is a way of making decisions without seeming to decide.” So the first response to data is to recall that data has a source, that it does not mysteriously come into existence untainted by human interests. The first response is to remind ourselves that data is created by institutions, which make decisions about what categories to use and what information to collect—and which to ignore.
Second, policymakers and data users should remember that not everything is as tractable to the methodologies of data. It is tempting to act as if data-based methods simply offer direct, objective, and unhindered access to the world—that if we follow the methods of data, we will banish all bias, subjectivity, and unclarity from the world. The power of data is vast scalability; the price is context. We need to wean ourselves off the pure-data diet, to balance the power of data-based methodologies with the context-sensitivity and flexibility of qualitative methods and local experts with deep but nonportable understanding. Data is powerful but incomplete; don’t let it entirely drown out other modes of understanding.
It’s not like qualitative methods are perfect; every qualitative method opens the door to other kinds of bias. Narrative methods open the door to personal biases. Trusting local, sensitive experts can open the door to corruption. The point is that data-based methodologies also have their own intrinsic biases. There is no single dependable, perfect way to understand or analyze the world. We need to balance our many methodologies, to knowingly and deliberately pit their weaknesses against each other.