A Silicon Valley Catechism
Review of
Machine, Platform, Crowd: Harnessing Our Digital Future
New York, NY: W. W. Norton & Company, 2017, 402 pp.
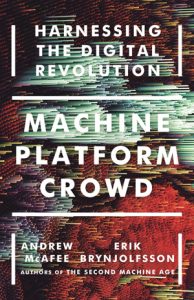
For over a decade, business books have exhorted managers to be “supercrunchers”—numbers-obsessed quantifiers, quick to make important decisions as “data driven” as possible. There is an almost evangelical quality to this work, a passionate belief that older, intuition-driven decisions are a sinful relic of a fallen world. With Machine, Platform, Crowd: Harnessing Our Digital Future, Andrew McAfee and Erik Brynjolfsson aim to formalize the successive canonizations of statistics, big data, artificial intelligence, and machine learning into a consultant-friendly catechism of what smart business leaders should do today. Chapter summaries deliver a snappy series of commandments; chapter-ending questions are designed to ensure readers (presumably managers and B-school students) have not missed the point.
For McAfee and Brynjolfsson, firms such as Google, Uber, Facebook, and Airbnb are the business models of the future. The authors emphasize certain secrets of success at such platforms: scale quickly, achieve user lock-in, and extract revenues by taking a cut of transactions linking consumers and advertisers or service providers. They gloss over tactics such as regulatory arbitrage and tax avoidance. Large platforms now command so many resources that their lobbying efforts can easily swamp those of fragmented and uncoordinated incumbent firms. Whether this is a feature of contemporary capitalism or a bug is left unclear.
To be fair, the authors have in prior work laid out an impressively comprehensive vision of a new social contract for an era of automation. They have advocated for a larger government role in educating workers for new, higher-skilled jobs and ensuring subsistence for those left behind by technological change. But this broad-minded, even magnanimous approach is not much in evidence in Machine, Platform, Crowd. Instead, we see advice aimed at accelerating the types of disruptive social change that their past work was more cautious about.
McAfee and Brynjolfsson’s work on platforms would be improved if they took critical voices more seriously. For example, consider platforms for labor such as Uber. Uber’s gig workers were among the first to realize that the vaunted flexibility offered by the sharing economy may just be a cynical rebranding of job insecurity and a lack of benefits—what some scholars have termed “precarity,” implying a precarious existence. Uber drivers found that their digital bosses were often taking a bigger and bigger cut of revenues, as their own needs for steadier work and benefits were ignored. Researchers exposed how misleading official pay figures were, because they didn’t include costs such as gas, insurance, or car payments.
Uber’s customers have also started to complain. Surge pricing seemed arbitrary. Sometimes the same ride would cost much more for one person than another. Creepy tracking and rating practices proved shocking. Next, the government woke up. Judges and regulators started to force Uber and other firms to recognize that they were employers, not just software providers. For example, regulators discovered that many Airbnb properties were stealth hotels owned by the very wealthy, not PR-friendly “mom-trepreneurs.”
The story of platforms is a lot less sunny than the narrative presented in Machine, Platform, Crowd, once their negative effects are fairly tabulated. Do we really want the kingpins of Uber and Lyft wielding outsized power over transportation policy, or Airbnb to further centralize room-letting and perhaps move into virtual property management? The authors dodge the hard political questions that arise as the bloom comes off the rose of platform capitalism and antitrust scholars criticize the centralization of power that accompanies winner-take-all digital markets.
Nor are the machines at the core of McAfee and Brynjolfsson’s whiggish narrative of business progress as infallible as they suggest. In their take, machine learning reigns over all business (and perhaps even government and nonprofit) functions as a master profession. But as the sociologist Will Davies has observed, “A profession that claimed jurisdiction over everything would no longer be a profession, but a form of epistemological tyranny.” And it is a confused tyrant at that. At points in their narrative, the authors denigrate human experts in general, cajoling top managers and investors to subject every person they rely on to the crucible of comparison with some type of automated prediction engine. Yet most business and financial experts today already rely extensively on computational systems. What the authors really seem to be promoting is an ever more intense standardization of business practice on algorithmic terms. Are robot CEOs the logical endpoint of their program?
In earlier iterations of artificial intelligence, researchers tried to reduce human expertise to a series of propositions, rules to be applied by an expert system. It turns out that although this approach can work well for very narrow applications, it is difficult to formalize human reactions and skills into a series of rules. That difficulty has not turned out to be an insuperable barrier to various schools of machine learning.
For example, with enough data and computing power, machine learning experts can try multiple algorithms to optimize performance. McAfee and Brynjolfsson mention the difficult problem of managing the temperature of a server farm, and it is easy to see how a computer program could solve the problem second-by-second better than any human expert because there are so many variables (airflow, temperature outside, computational intensity in various parts of the building, and so on) that need to be computed nearly instantaneously. Moreover, a cutting-edge system can experiment, shifting allocations of cooling effort among, say, fans, air conditioners, and other methods, or determining whether a relocation of computing activity (toward, say, colder walls in winter) might be more cost-effective than increasing airflow in areas prone to overheating.
Various machine learning methods are now being developed by different schools of computer scientists. Basic pattern recognizers can map a classic response to a given situation. Evolutionary algorithms can spawn a large number of approaches to a problem, experiment with them, and determine which one works best, ready to be deployed in the future. Bayesian classifiers can weigh evidence about whether a given strategy is working or not, modeling causation along arcs connecting different nodes in a network. And some programs even compose approaches on the fly, coming up with the types of nonhuman intelligence that wowed commentators during the victory of AlphaGo, Google’s artificial intelligence program for playing this complex Chinese board game, against the reigning Go champion in 2016.
McAfee and Brynjolfsson begin Machine, Platform, Crowd with the story of AlphaGo, and quickly parlay it into a metaphor for an eventual, general advantage of machine learning driven approaches over human judgment. They contrast machines’ implacable, objective data analysis with humans’ tendency to distraction and subjective judgments. We finally know whether humans can win the “race against the machine” (the title of the authors’ first book together): beset by cognitive biases, they are no match for algorithmic decision making. McAfee and Brynjolfsson mock the usual business decision making as the mere opinion of the “Highest Paid Person in the Organization” (HiPPO); the evocation of a dumb, clumsy, oversized creature fated to be subdued by technology adds a frisson of rebellious cheekiness to their program.
Unfortunately for any manager looking to this book as a turnkey solution to strategy, the authors’ case for machine learning is overstated—even self-contradictory. To suggest that software can be optimized to make better decisions than humans, they offer a series of examples to demonstrate weaknesses in human judgment. A sociology professor used a mathematical model to predict firms’ adherence to budget and timeliness of product delivery better than purchasing managers. A county’s nonverbal IQ test included more minority children in a gifted program than a process centered around parent and teacher nominations. Law professors’ simple, six-variable model predicted Supreme Court rulings for the 2002 term better than 83 prominent legal experts did. From examples such as these, and a simple behavioral economics story about human susceptibility to instinctual rashness, the authors conclude that “The evidence is overwhelming that, whenever the option is available, relying on data and algorithms alone usually leads to better decisions and forecasts than relying on the judgment of even experienced and ‘expert’ humans.”
But where do the algorithms and data come from? As digital sociologist Karen Gregory has observed, big data is made of people. People develop algorithms to parse it. People are part of the “crowd” that McAfee and Brynjolfsson (following Clay Shirky’s Here Comes Everybody) praise for supplying data and labor to so many machine learning applications, in such diverse areas as spam detection and targeted ads. Sophisticated work in critical algorithm studies repeatedly emphasizes the intertwining of computational and human elements in decision making. So why are the authors so intent on maintaining an outdated dichotomy?
Even more damningly, the parade of examples they give of “superior” automated decision making are themselves no more than a narrative of computational supremacy. They give no sense of the universe of studies available on the comparative advantage of computation over human decision making, the applicability of these studies, or even whether their examples have been replicated or reanalyzed. Without grounding in such basic statistical concepts, their sweeping claims (one study on Supreme Court prediction is a clue to the future of the entire legal industry! One logistics model could eliminate vast swathes of human labor in that field!) will ring hollow to anyone with even the slightest critical faculty.
Of course, this probably will not be too great a commercial problem for McAfee and Brynjolfsson, since the most likely function of their book is to help managers justify workforce reductions and pay cuts. Like the management consultants brought in to offer a post hoc imprimatur for managerial decisions made long before, the current deluge of “creative destruction” dogma is a tool of rationalization in the Freudian, not Weberian, sense. But even the most cutthroat managers should think twice before hitching their wagon to the stars of machine learning. Not only is it “remarkably easy to incur massive ongoing maintenance costs at the system level when applying machine learning,” according to a group of Google researchers—it is also often hard to determine whether one has the right training data set even to begin a machine learning process. Furthermore, will strategies derived from past data still work? Perhaps we can apply machine learning to past machine learning efforts to find out, but given the proprietary status of both algorithms and data there, I would not hold my breath for that recursive strategy.
Advanced predictive analytics can also easily become a tool of discrimination. The all-too-human elements of machine learning were recently on display in an extraordinarily troubling paper by Chinese researchers titled “Automated Inference on Criminality using Face Images.” The authors used a machine learning algorithm, trained on a data set of faces of roughly 2,000 criminals and noncriminals, to “empirically establish the validity of automated face-induced inference on criminality, despite the historical controversy surrounding this line of enquiry.” They even provided four archetypal images of “criminal” faces. Critics denounced the study as a rationalization of discredited theories of caste, phrenology, and the innate inferiority of some human types. But the study authors stood firm, defending the importance and validity of their findings.
The facial criminality study raises tough questions for McAfee and Brynjolfsson. At the end of their cursory discussion of algorithmic discrimination, they try to defuse concerns by offering reassurances that machine learning systems can be corrected. (And their point is an even stronger one—that these systems are far more capable of being corrected than humans.) But aren’t there some machine learning projects that are simply too invasive or unethical to conduct? A firm now sells facial analysis technology to assess a person’s health status. Should a retailer use that software to figure out which customers to shun because they will likely be too ill to purchase much soon? Should it use the software on employees or job applicants?
Critical thinkers may also suspect that algorithmic reasoning can fail on its own terms. McAfee and Brynjolfsson praise Barack Obama’s presidential digital campaign team, but then explain away Hillary Clinton’s loss by suggesting that the “quality of data inputs” for her campaign’s analytics system, Ada, were flawed. But that kind of just-so story makes the authors’ claims about predictive analytics unfalsifiable: if they ever falter, low-quality data can take the blame. The hard questions arise in real time; Monday-morning quarterbacking can’t be taken seriously unless the authors specify what outcome would lead them to second-guess their preferred methods of prediction and management.
In McAfee and Brynjolfsson’s telling, predictive analytics emerges less as a way to ensure that current decisions are right and more as a method of organizing information to ensure better decisions in the future. They apparently believe that the tool is destined to become the prime method of improving business processes. But as long as free will and capacities for self-expression endure, the “crooked timber of humanity,” to borrow a line from the philosopher Immanuel Kant, will offer opportunities to resist the method’s rationalizing bent—and smart managers, whether of campaigns or businesses, will be cautious about biases, inconsistencies, and gaps in the data they use.