Beyond Patents
Scholars of innovation use patenting as an indicator of both innovativeness and the value of science. It might be neither.
Over the past 20 years, patent analysis has become a dominant method of studying innovation. The number of papers containing “patent citation” listed in Google Scholar rose from fewer than 10 in 1998 to more than 900 in 2014. For papers published in seven journals of the American Economic Association since 2010, 9 of the 20 judged most relevant to innovation by the association’s search engine used patent analyses. If papers that analyze sociolinguistic, behavioral, consumer sentiment, and measurement innovations are excluded, to focus exclusively on product, process, and service innovation, the proportion rises to 9 of 16. For Management Science, the leading management journal on innovation, 11 of its 15 articles most relevant to innovation and published since 2010 used patent analyses.
For many of these papers, the focus is on the relationship between innovation and advances in science. Patents themselves are used as a measure for innovation, whereas papers cited in the patent applications are used as a measure for advances in science. For example, Science, the world’s leading generalist science journal and one that publishes few social science papers, did so in August 2017 with an article, “The dual frontier: Patented inventions and prior scientific advance.” This study concluded that “most patents (61%) link backward to a prior research article” and “most cited research articles (80%) link forward to a future patent.”
Questions about these types of analyses have been asked since long before they became the dominant method of analyzing innovation. Strengths and limitations of patent analyses as indicators of innovativeness and scientific advance—such as the fact that not all inventions are patentable and not all innovations are patented—have been thoroughly reviewed over the past few decades by scholars of innovation such as Zvi Griliches and Martin Meyer. But the mixed value of patent analysis seems to have had little influence on the growing use of patent data by those studying innovation and science. In my view this growing influence of patent analysis reflects a fundamental bias in the social sciences toward large-scale empirical analyses of quantifiable databases aimed at uncovering allegedly general economic principles, and away from studies that can reveal the complexity, context, and processes of technological innovation in the economy.
I argue that the growing dominance of patent analysis not only fails to provide valuable and reliable insight into innovation processes, but is a smoke screen that prevents social scientists and policy-makers from understanding real problems and processes of innovation. Further, excessive focus by innovation scholars on studying patents, and by academic scientists on pursuing patents, together suggest the possibility that increased patenting activity may contribute to a long-term slowdown in productivity growth. We need an alternative research agenda for studying the relations between science and innovation, and I propose the outline of such an agenda with a focus on productivity.
What good is patent analysis, and what good are patents?
It has long been recognized that most innovations are not patented and that many patents don’t represent important innovations. There are many reasons for this. One is that most innovations represent a combination of ideas, some of which represent novel patentable designs and some of which do not. Another reason is that the benefits from patenting do not exceed the costs for many types of innovations, and thus firms do not apply for patents. Patent law offers patent recipients protection in return for disclosure, but not all innovators benefit from this trade-off. The ones who do not apply for patents do not have their innovative activities counted as innovations in patent analyses, even though many of their activities are highly beneficial to the users.
For example, consider the Wall Street Journal’s “billion-dollar startup club,” new firms that are valued at $1 billion or more. These start-ups can certainly be defined as innovative, even if their values are probably inflated. Table 1 shows the percentage of start-ups by numbers of patents (as of February 1, 2018). Only 41% of the 170 start-ups had at least one patent and only 20% and 9.4% had at least 10 and 50 patents, respectively. The percentages are particularly low for e-commerce, financial services (fintech), and consumer internet, categories that include highly valued services such as ridesharing and room sharing, e-commerce for fashion, social networking, peer-to-peer loans, and mobile payments. Patent analyses miss these types of innovations and thus represent a highly skewed view of innovation in the US economy.
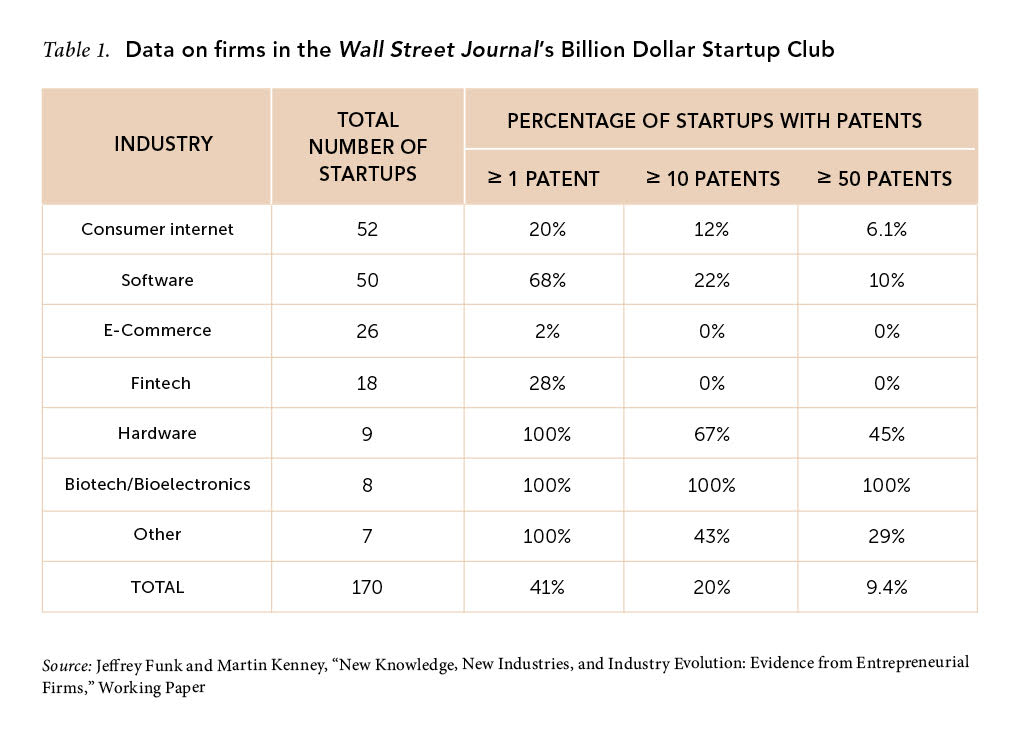
What economists really want to understand about innovation is its impact on productivity, because in the long run productivity growth is the most important issue for economies. Economists have long been aware that there is little correlation between total factor (or labor) productivity and total patenting numbers. This led early researchers on innovation such as Jakob Schmookler to recognize as far back as the 1960s that patents were a better index of innovative “activity” than of the actual economic output from this activity. He was concerned with what patents can measure rather than what we would want them to measure, a lesson that many innovation scholars have forgotten.
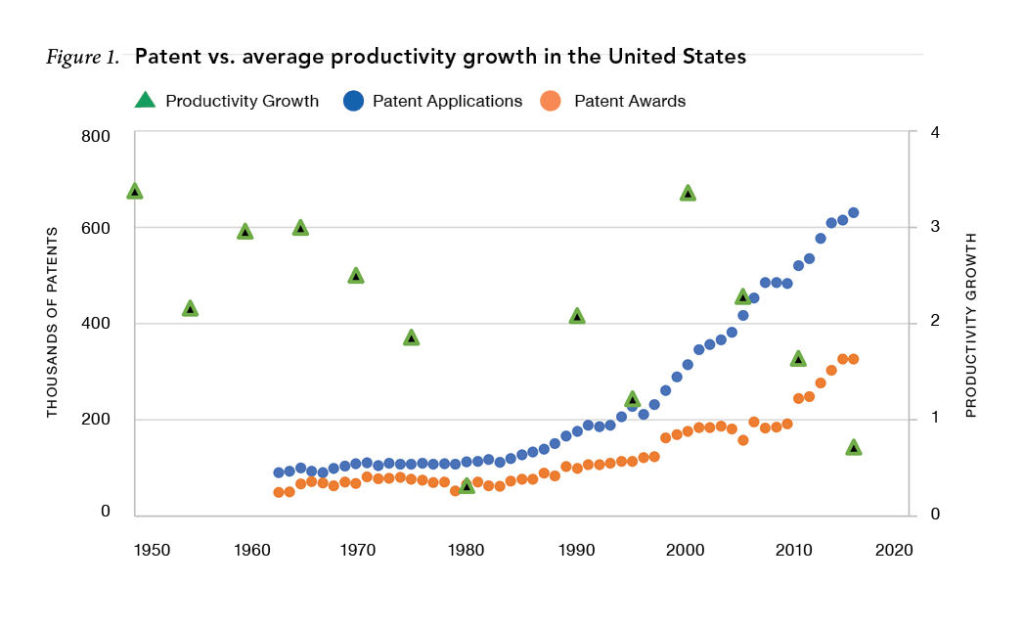
Figure 1 plots the number of patent applications, patent awards, and average productivity growth (five-year averages) over time. The numbers of US patent applications and awards were flat until the mid-1980s, when patenting activity began its current period of explosive growth. First noted by the economist Brownyn Hall in 2004, patent applications increased by more than three times between 1984 and 2003 and more than six times by 2015. Patent awards also rose by almost six times between 1984 and 2015. Reports from the World Intellectual Property Organization show similar trends; global patent applications quadrupled between 1980 and 2015 while global licensing income from patents grew even faster, rising by more than five times between 1980 and 2009 and by three times in terms of percent of gross national product (GNP).
Figure 1 also shows that US productivity growth has not increased along with patent applications and awards, suggesting that patents are not a good measure of innovation. If patenting activities were a good measure for innovation, productivity growth should have also increased, probably with some type of time lag. However, growth in US productivity has slowed since 1970, and that slowing is in addition to the slowing that has occurred since 1940. Other than short-term decreases (1980) and increases (2000), the average productivity growth after 1984 is not higher than before 1984. Furthermore, Anne-Marie Knott of Washington University has found that corporate research and development (R&D) productivity has been falling as well, with corporate revenues generated per dollar of corporate R&D spending having dropped 65% in the past 30 years, even as patent applications and awards have exploded. Understanding the reasons for a slowdown in economic productivity growth and a fall in R&D productivity should be major goals of the economics discipline, but don’t expect much from patent analyses.
Looking at Figure 1, it is tempting to advance a hypothesis that patent applications could be one reason for the lack of productivity growth. In addition to patents slowing the diffusion of information, could patent applications be a distraction for engineers, scientists, and their managers because they require large amounts of administrative work in applications and infringement cases? Since the cost of applying for a patent is about $10,000, it is not hard to imagine that if the cost of engineers, internal lawyers, and other personnel are included, the cost could easily reach $50,000 per patent. With 630,000 patent applications in the United States in 2015, this suggests the US market for patent activities that year was about $30 billion, equivalent to about half of the entire federal investment in nondefense R&D. Since economists have noted the negative impact of environmental, health, and safety regulations on productivity growth, why wouldn’t they take seriously the possibility that the administrative work of patent applications distracts the nation’s engineers and scientists, including those at universities, from the real work of innovation?
Evidence for the wastefulness of patenting can be seen in the lack of correlation between patenting and market capitalization (i.e., profitability) in Table 2. Researchers have long recognized that patents have little impact on firm profitability, and this problem still exists. The World Intellectual Property Organization reported in 2016 the top 100 organizations (both firms and universities) in terms of patent applications for four recent years (2010-2013), and PwC listed the top 100 in terms of market capitalization as of March 30, 2013. Of the top 10 patent applicants, only three were in the top 100 for market capitalization: IBM was ranked 9th, Samsung was 19th, and Toyota was 27th. Expanding the leading patent applicants to the top 100 adds only six more from the top 100 market-capitalized companies, for a total of nine companies. Looking the other way, only four of the top 10 companies for market capitalization were among the top 100 patent applicants, and they were ranked 9th (IBM), 31st (GE), 45th (Microsoft), and 65th (Google). Apple, Exxon Mobil, PetroChina, Walmart, and Nestle are not even in the top 100 even though many of them are in industries that do apply for many patents.
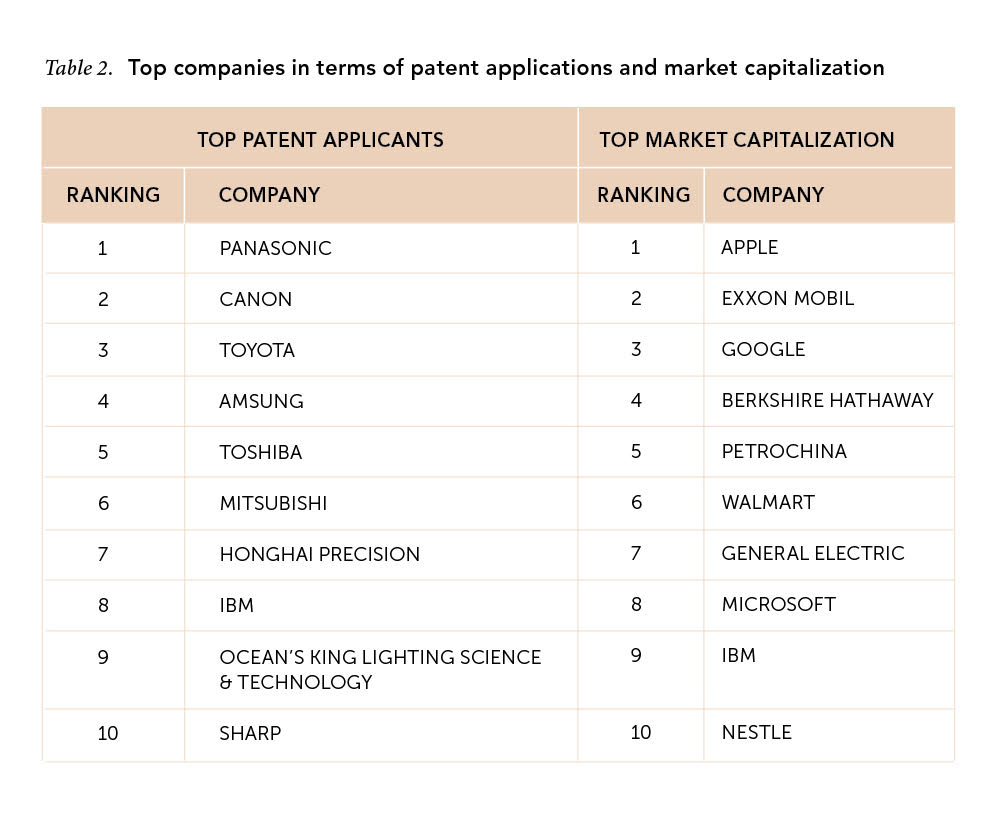

Proponents of patents would argue that patent citations are a better predictor of profitability because they show which patents are viewed as important by other innovators, and thus Table 2 should rank firms by the number of citations to their patents, and not the number of their patent applications. But such logic calls to mind the old adage (variously attributed to Mark Twain, Niels Bohr, and Yogi Berra) that “prediction is hard, especially about the future.” Patent citations are the same. In retrospect one can identify the important patents, but beforehand most of us have great difficulty knowing which patents will end up being heavily cited. So using patent citation to show that patents are important indicators of profitability is circular. If top companies aren’t patenting much, they must not think that patenting is important for profitability.
These observations suggest that scholars of innovation need to take seriously not only the possibility that patents are not important predictors of innovativeness, but that the increasingly intense focus by firms and universities on patenting may be distracting them from more important work.
What can patents tell us about science?
As in the recent Science paper cited above, many patent analyses treat citations of science papers in patent applications as a measure of knowledge flows. Yet scholars have long known that citations of science and engineering papers in patents are not a very good measure of knowledge flows, or of advances in science, and that informal interactions between and among university researchers and corporate engineers may be more important. Patent citations do not reflect the actual activities or thought processes of the engineers and scientists who devise novel designs and submit patents. Instead, they reflect the efforts of many participants in the patent application process to distinguish new ideas from prior ideas, including prior patents and public information. Such efforts amount to a complex sociopolitical dance among applicants and patent examiners to determine the scope of patents. Specialist patent advisers, not the scientists and engineers themselves, are necessary because expanding the scope of a submitted patent while narrowing the scope of competing patents requires a special set of skills. The patent examiner then tries to understand the novelty and limits of the claimed versus competing inventions. The result is that both patent examiners and advisers add many of the papers eventually included in patent applications, whereas the engineers and scientists who did the work may not have read many of the papers, or even have been aware of them.
This process was well illustrated in a detailed study of patent applications by Martin Meyer, who used interviews with scientists and engineers to better understand where their ideas came from. The study looked at patents for nanoscale technologies, an area of innovation that probably benefited from advances in science more than most technologies, yet the study concluded that few of the ideas came from academic papers, but rather from the independent work of the engineers and scientists. Many of the papers added by the patent examiners could not be recognized by the scientists and engineers who devised the novel ideas for the patent applications.
Nor are counts of citations of papers in patents a good measure for advances in science. For example, the types of journals cited in patents include not only scientific journals but also engineering and even management journals. Patent citation studies typically use the Science Citation Index (SCI) or the Web of Science (which includes the SCI) to identify science journals, but the SCI includes not only science journals but also engineering and business journals; even Harvard Business Review is included in the index. Including engineering journals in the list of journals used to count scientific papers overestimates the contribution from scientific journals. In a previous article in Issues (Spring 2017), I looked at 143 members of the billion-dollar startup club and found that most of the papers cited in their patents were from engineering journals published by groups such as the Institute of Electrical and Electronics Engineers or the Association for Computing Machinery, and not basic science journals such as Nature and Science, nor standard disciplinary journals. More specifically, although 18% of the 143 start-ups had patents that cited at least one paper in the SCI, only 6% of them had patents that cited a scientific as opposed to an engineering journal. Lumping engineering papers together with basic scientific papers as a surrogate for advances in science so generalizes the phenomenon of “knowledge flows” that it thoroughly undermines the value of that concept for assessing the relations between scientific advance and innovation.
I am arguing that research on patent databases may not help us understand innovation. But I am also suggesting that such research may rather be a smoke screen, hiding the true issues, problems, and dynamics of innovation behind an illusion that innovation is booming and that rising scientific and patenting activities are the reason for the boom. Many innovation scholars seem to believe that patents are more important indicators of innovation than are new products, services, and processes and their contribution to productivity improvements and company profits. Although some highly cited patents are of course extremely important and valuable, and some scientific papers are very influential, the patent obsession makes us think that such cases are everywhere, and are what drive an innovative economy, when in fact “home runs” such as transistors are rare.
This type of research is also self-serving, placing academics at the center of the economy while ignoring the other sources and forces behind new products, services, processes, and the entrepreneurs that introduce them. It enables university professors to claim that they are doing what they are supposed to do: writing papers that are cited in patents. It enables Science magazine to claim that it is the driver of innovation and productivity growth. More generally speaking, the emphasis on patents and papers reflects a much larger problem in the social sciences: an increasing obsession with large databases, sophisticated statistics, and elegant mathematics. This quantification arms race has pushed and continues to push the social science research on innovation toward more analyses of large patent databases, yet it may also be pushing it away from true understanding of how innovation works in today’s complex and varied economic settings. In part this is a classic problem of looking for one’s lost keys under the streetlight. In this case, if the keys are the complex dynamics of science, innovation, and productivity, the streetlight is patent data, and what keeps researchers looking in the same place is the academic incentive system, where publications are the sources of promotions, job security, social and intellectual status, and pay increases.
A new agenda for innovation research
If patent analysis is a smoke screen that has prevented scholars from improving their understanding of innovative processes, how should they address innovation instead? Although there are many possible alternative avenues, here I focus on a new agenda for productivity that is being advocated by a small number of scholars such as Robert Gordon and Tyler Cowen, but is largely neglected by most innovation scholars. A first set of questions involves the extent to which new products, services, and processes have emerged and are currently emerging. GNP data tell us some of this, but not anywhere to the extent that is needed. We need databases that better highlight these changes, particularly in the early years of new products, services, and processes. This includes science-based technologies such as superconductors, quantum computers, nanotechnology, synthetic food, glycomics, and tissue engineering; new forms of digital products such as augmented reality and drones; and new forms of internet services including those that are free, such as music and user-generated content.
Without data on recent products, services, and processes, it is difficult to understand what has emerged in the past 20 to 40 years, the period that is the most important to analyze. Fine-grained sales data from this and previous periods can help us understand the extent to which new products, services, and processes have been emerging and help us analyze the impact of changes in regulatory policy and in university and government R&D policy on the emergence of new products, services, and processes, particularly recent ones. A possible source of data is the growing number of market research organizations that provide regularly updated data on new technologies. The challenge is to integrate these data with traditional GNP data because this integration requires a different set of skills. Scholars must understand the technologies that form the basis for the new products, services, and processes, a research activity that is very different from statistically analyzing large databases.
Second, once we know what is coming out, we can address where these new products, services, and processes come from. Do they come from existing technologies or new technologies? If they come from existing technologies, what enabled them to emerge when they did? Was it changes in regulation, consumer demand, or cost and performance of existing technologies? If they are from new technologies, how did these new technologies emerge? What were the changes in cost and performance that enabled them to emerge, and were there advances in science that formed the basis for the new products or that helped the improvements to occur? Addressing these questions will help us understand the overall processes that lead to the emergence of new products, services, and processes, along with the factors that influence the processes. Currently, there is little agreement about where new products, services, and processes come from, a seemingly basic issue.
Third, researchers should be looking the opposite way. How are the new types of products, services, and processes that are economically important today connected to the many types of scientific research that have been funded over the past 20 to 40 years by the National Science Foundation (NSF), the National Institutes of Health, and other government agencies? Most funding agencies and analyses of this research merely focus on academic papers as an output, but what matters is the research that eventually leads to new products, services, and processes. This analysis should go beyond the standard litany of anecdotes (by now everyone knows that the Google algorithm was developed by NSF-funded researchers at Stanford University) and be able to trace the linkages between specific advances in science and the eventual products, services, and processes, including the intermediate steps of new product concepts and improvements in the cost and performance of the resulting technologies.
In a blog that accompanied the above-mentioned Science paper, the authors illustrated the importance of indirect linkages between science and technology by invoking the necessity of Einstein’s theory of relatively for the Global Positioning System and thus Uber. But such high-level, general linkages to paradigm-busting geniuses such as Einstein tell us almost nothing useful about knowledge flows, innovation, or science policy options. Einstein’s theory of relativity was published in 1916. What we need to know is the extent to which recent advances in science affect new products and services. We already know that most of the world’s products and services depend on past advances in science and that these advances are cumulative. We want to know how many advances made since 1980, 1990, or even 2000 have had a major influence on new products and services. This type of data is needed to understand the bottlenecks for innovation.
Patent analyses suggest that advances in science have been making direct contributions to every new product and service, but this conclusion seems unlikely given the successive productivity slowdowns in the US economy since 1970, even as government support for academic basic research increased more than tenfold (after inflation) between 1950 and 1980. These increases in basic science should have led to a productivity boom in the late twentieth century, one that rivals the late nineteenth century. Techno-optimists might argue that the boom is coming soon, but we need better data and analyses to test such a hypothesis. Among other things, we need such data to analyze the effects of various policies (such as the Bayh-Dole Act of 1980 that incentivized university patenting) on the linkages between advances in science and new products, services, and processes. Linking advances in science with real products and services requires a completely different form of research than is currently done in patent analyses. Rather than do large-scale empirical analyses, one must understand many intermediate linkages through detailed case studies. What types of new explanations did these advances entail? What types of new concepts or what types of performance and cost improvements in the resulting technologies did the explanations enable? What types of products, services, and processes emerged from these technologies, and what were the time lags? This type of research changes the focus from statistical analysis that searches for perhaps nonexistent general trends, to a real-world, case-based understanding of science and the emergence of new products and processes, such as the work pioneered decades ago by innovation scholars such as Kenneth Flamm on computers, Nathan Rosenberg and David Mowery on aircraft, Yujior Hiyami and Vernon Ruttan on agriculture, and Richard Nelson on transistors.
We also need to understand from which scientific disciplines the new products, services, and processes have emerged if we are to make better funding decisions and if we are to help engineering and science students make better career decisions in an ocean of hype about the value of science, technology, engineering, and mathematics (STEM) education. The current system makes little attempt to help students understand what types of innovations are occurring, what fields of science are making the most useful contributions to innovation, and thus which courses of study offer the most opportunities.
A fourth set of questions revolve around why some sectors have faster productivity growth than do others. Because most economic and management research focuses on organizational factors such as employee performance measures, incentives, and skills, and uses patents as a surrogate for innovation, the reasons for the differences between sectors is largely being missed. For example, the mechanics of Moore’s Law, the role of smaller scale, and the impact of this smaller scale on rapid improvements in cost and performance of information processing technologies are well documented, but little systematic effort has been made to look across industrial sectors and classes of technology to understand the overall impact of Moore’s Law and new materials on differences in productivity, or to identify other specific pathways of innovation on productivity. Patent analyses gloss over these details and leave us with a vague feeling that innovation is occurring, science supports this innovation, and as long as we have more of both, everything will be okay.
Innovation scholars should be trying to better understand the reasons for the productivity slowdown and how it can be fixed. Identifying and analyzing these reasons will require scholars to consider multiple types of data and information, much of which cannot be placed in a spreadsheet and analyzed with sophisticated statistics, and will not likely be found in academic journals. Scholars will have to get their hands dirty, understanding the specifics of new advances in science, new technologies, and their resulting new products and services. They will have to make judgments, create new definitions, identify new linkages, and begin building new bodies of data. Just as Charles Darwin left home to understand the real world, innovation scholars need to do the same, leaving the safety of existing databases and theories so that they can start collecting new data and generating new hypotheses. Ultimately, such research can help inform a more constructive discussion about fostering economic opportunities across all levels of society.