Bringing Communities In, Achieving AI for All
To ensure that artificial intelligence meaningfully addresses social inequalities, AI designers and regulators should seek out partnerships with marginalized communities to learn what they need from this emerging technology—and build it.
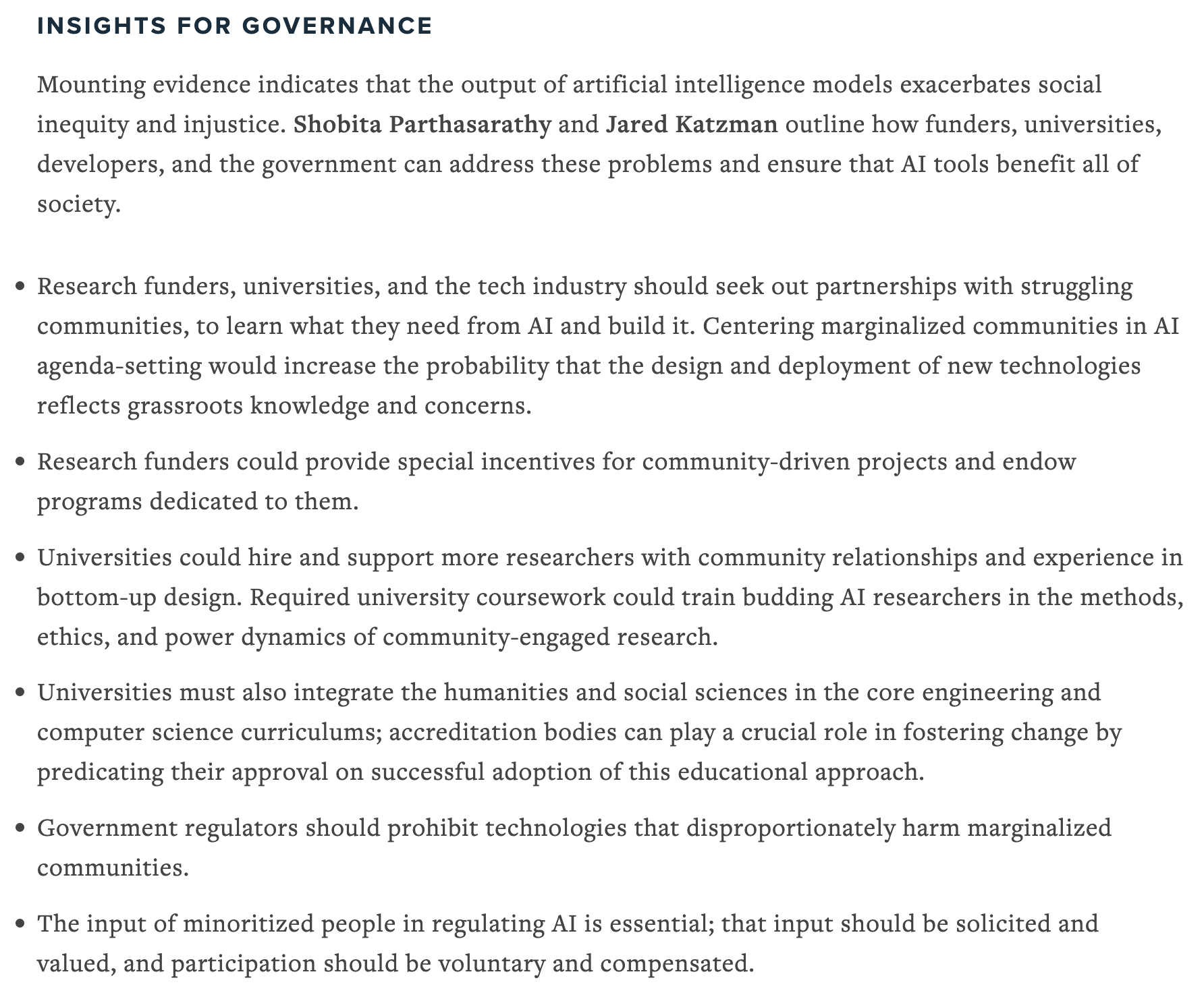
Hopes for the future of artificial intelligence would seem to be bright. There is, after all, a great deal of utility in AI already. AI tools can provide high-quality real-time translation for well-resourced languages like English—a major technological breakthrough. AI systems also are poised to enhance the accuracy of cancer screening and improve other areas of health care delivery.
Yet much of the discourse surrounding AI is rather gloomy. It’s not just that people worry about possible effects of generative AI on their livelihoods; innovation-driven employment disruptions are neither unusual nor insurmountable. More concerning is the mounting evidence showing that the output of AI models exacerbates social inequity and injustice.
Facial-recognition technology, famously, is proving to be a tool of oppression, as many have feared. Reports of AI triggering false arrests of Black people are becoming routine. Municipalities are using facial-recognition cameras to aggressively surveil and police residents in public housing, many of whom are Black. Against hopes that AI would reduce bias in criminal justice, its use so far has magnified the system’s structural inequalities. Meanwhile, major AI firms like OpenAI are exploiting overseas sweatshop labor to train algorithms. And AI tools meant to benefit people with disabilities are having the opposite effect. This situation is creating real harm for people who are already disadvantaged, while also amplifying distrust in science and government.
Proposed responses to these equity and justice concerns typically amount to small tweaks, often of a technical nature. The thinking seems to be that policymakers, academics, and the technical community can solve AI’s problems by identifying statistical biases in datasets, designing systems to be more transparent and explainable in their decisionmaking, and exercising oversight. For instance, experts ask how government agencies might evaluate the safety and efficacy of algorithms. In parallel, the technology industry has tried to educate developers about the impact of social biases on AI algorithms and has suggested minimal “fairness solutions” also focused on bias.
We must ask ourselves if we really believe that marginalized people should be content to leave their fates to the tinkering of governments and corporations when such measures have had little impact in the past. Where is the input, in this equation, of marginalized people themselves? If we are concerned by equity in the age of AI, shouldn’t those with the most at stake have an important role in shaping the governance agenda? Then too, relying only on governance by state authorities and commercial operatives means ratifying and reinforcing the concentrations of economic and political power that already accrue to a small number of well-connected businesses. Their technical revisions to the mechanics of AI may address some harms built into the technology so far but will always be behind the curve of inequities that emerge as AI makers exercise, and strive to protect, profit-seeking prerogatives that inevitably displace their stated commitments to just outcomes. And simply extending regulatory oversight does not encourage developers to design AI that promotes the welfare of the disadvantaged.
Technical revisions to the mechanics of AI may address some harms built into the technology so far but will always be behind the curve of inequities that emerge.
Better solutions lie in a more inclusive innovation ecosystem in which all players—not just regulators and lobbyists—take responsibility for creating equitable and just AI. It is important that AI not only not discriminate but also that it be proactively marshaled to the benefit of all of society. In other words, we should be thinking about how to ensure that AI is not just here for profit but also to serve those who, in being served, don’t generate financial returns.
To this end, public and philanthropic research funders, universities, and the tech industry should be seeking out partnerships with struggling communities, to learn what they need from AI and build it. Regulators, too, should have their ears to the ground, not just the C-suite. Typical members of a marginalized community—or, indeed, any nonexpert community—may not know the technical details of AI, but they understand better than anyone else the power imbalances at the root of concerns surrounding AI bias and discrimination. And so it is from communities marginalized by AI, and from scholars and organizations focused on understanding and ameliorating social disadvantage, that AI designers and regulators most need to hear.
A Community Agenda for AI: Design from the Bottom Up
Progress toward AI equity begins at the agenda-setting stage, when funders, engineers, and corporate leaders make decisions about research and development priorities. This is usually seen as a technical or management task, to be carried out by experts who understand the state of scientific play and the unmet needs of the market.
But do these experts really understand which needs are unmet? When experts steer innovation, they are deciding which problems are important and how they should be understood and solved. Often, the problems deemed important are those that, in being addressed, yield profit. But sometimes developers try to solve social problems, usually with minimal input from the populations most affected. This leads to misdiagnosis. Such is the story of the One Laptop per Child program, for instance. Developed by the MIT Media Lab and funded by international donors, the initiative was supposed to improve education for children from low-income families around the world by ensuring that the children had access to internet-connected computers. But the project failed because the computers were not easy for the children to use, broke frequently and were difficult to repair, and relied on electricity that was at best intermittently available. Even when the computers worked, the content built into them contributed little to the realization of local educational goals.
When experts steer innovation, they are deciding which problems are important and how they should be understood and solved.
Centering marginalized communities in AI agenda-setting would help to avoid such outcomes by increasing the probability that the design and deployment of new technologies reflects grassroots knowledge and concerns. This approach may be slower and harder to scale than technology development based solely on expert opinion, but it is more likely to produce social benefits.
A heartening example comes from Carnegie Mellon University, where computer scientists worked with residents in the institution’s home city of Pittsburgh to build a technology that monitored and visualized local air quality. The collaboration began when researchers attended community meetings where they heard from residents who were suffering the effects of air pollution from a nearby factory. The residents had struggled to get the attention of local and national officials because they were unable to provide the sort of data that would motivate interest in their case. The researchers got to work on prototype systems that could produce the needed data and refined their technology in response to community input. Eventually their system brought together heterogeneous information, including crowdsourced smell reports, video footage of factory smokestacks, and air-quality and wind data, which the residents then submitted to government entities. After reviewing the data, administrators at the Environmental Protection Agency agreed to review the factory’s compliance, and within a year the factory’s parent company announced that the facility would close.
Bottom-up design, in Pittsburgh and elsewhere, requires openness and humility from researchers, recognition of community expertise, and a desire to empower marginalized people. It means that the technical interests of engineers and the profit motives of corporations take a backseat to public interest: the needs of communities determine what, if anything, gets built. Researchers must be willing to cede authority to others who might be able to better serve community concerns. This approach not only helps to meet real needs but also fosters trust in science and technology among populations subject to mistreatment and neglect.
This approach may be slower and harder to scale than technology development based solely on expert opinion, but it is more likely to produce social benefits.
Processes like the Pittsburgh collaboration are unusual, typically initiated by technologists committed to community-driven research practices and by interdisciplinary teams of technical and social-science experts. But the institutions that support innovation can take steps to encourage bottom-up design. Research funders could provide special incentives for community-driven projects and endow programs dedicated to them. Universities could hire more researchers with community relationships and experience in bottom-up design and could provide these researchers additional support. Perhaps most importantly, required university coursework could train budding AI researchers in the methods, ethics, and power dynamics of community-engaged research. We believe that such training must start early—it should be foundational to the education of the next generation of AI innovators, so that they will not be content to simply follow in the footsteps of others but will instead be partners in transforming the AI innovation ecosystem.
Nurturing Socially Committed AI Research
As presently constituted, the AI industry is ill-equipped to respect the knowledge of marginalized communities. AI leaders, in business and academia, are a demographically narrow group, little influenced by public interest. Programs dedicated to social responsibility are treated as auxiliary rather than mission-critical and are the first to go when companies and universities cut budgets.
How to ensure that the current generation of technologists is the last to operate in this way? How to inculcate in engineers—and their leaders—a genuine interest in AI’s humanitarian benefits and greater sensitivity to its potential harms?
Required university coursework could train budding AI researchers in the methods, ethics, and power dynamics of community-engaged research.
One answer—though not the only one—is education. Universities can revamp the way they teach engineering, integrating the humanities and social sciences in the core curriculum. Today universities enforce a clear separation between engineering and the social good; they may require that STEM students take a single course on professional ethics, while the rest of the curriculum teaches students that technology is politically and morally neutral. More useful would be to design introductory science and engineering courses that help students understand the social and political assumptions underlying seemingly technical choices, as well as the consequences of these assumptions. For instance, when computer science students learn how to build datasets that inform an AI, they should learn at the same time that the contents of datasets—because they are based on historical records—could reflect racist practices.
Because it is essential that future AI researchers be empowered to reckon with the true social complexities of their work, humanizing education should be treated as no less important than technical education: it cannot be ghettoized in elective courses, where it is easily dismissed. Students must learn that technical decisions about research problems, datasets, and code are always value-laden. And humanists and social scientists must teach this lesson: it is they who offer deep knowledge of how technology works in society. Students need this knowledge, and they need intellectual models who disrupt the idea that only scientists and technologists have valuable expertise to offer in the development of AI. Accreditation bodies can play a crucial role in fostering change by predicating their approval on successful adoption of this educational approach.
The project of changing AI innovation through education should begin as soon as possible, but its rewards will take many years to realize. In the short term, there are opportunities for improvement through policy. As suggested by the case of Timnit Gebru, who says she was fired from Google for exposing deleterious ethical and equity consequences of the company’s software, AI development would benefit if researchers had stronger whistleblower protections. And funding agencies can support more socially conscious approaches right now, even requiring researchers to write proposals that champion the equity benefits of their projects. Doing so would encourage the most promising kind of AI development—development that does real good for all of society, including and especially underserved communities.
Building Capacity in Communities
Community participation is important not only because it can enable codesigned technologies like the Pittsburgh air-pollution system, but also because it fosters democratic engagement in decisionmaking surrounding emerging technologies. Whether these are decisions made by private companies or public officials, the people affected should have a say in them. Civil society organizations have a crucial role to play here, by amplifying voices drowned out by the industry din. Tech companies have effectively unlimited resources, as well as access to political power. To be heard, ordinary people need civic organizations to be their advocates, critically evaluating industry claims, anticipating the social effects of AI that corporations ignore, and using their own lobbying capacities to focus the attention of policymakers and regulators on the public good.
Humanizing education should be treated as no less important than technical education: it cannot be ghettoized in elective courses, where it is easily dismissed.
The Ford Foundation has been exemplary in this regard. The philanthropy funds multiple organizations that seek to improve the public conversation about AI and generate policy action. These include Fight for the Future and the Detroit Community Technology Project, which advocated for regulation of facial-recognition tools after Joy Buolamwini, a Black computer scientist, identified systemic biases in existing technologies. Other philanthropies have joined, and could still join, Ford in supporting nonprofits dedicated to the independent assessment and democratic control of AI.
Civic organizations have a further important role in providing bridges between communities and technologists by helping engineers and regulators understand AI through a social lens. Civil society, in other words, can do in the wider world what educators can do in the classroom, explaining how design can inadvertently exacerbate social problems and how it can be used with the goal of improving people’s lives. The University of Michigan’s Science, Technology, and Public Policy Program, for example, has established a Community Partnerships Initiative that serves this bridging function by working with advocacy organizations to develop proposals for technology policy in the public interest. For instance, the initiative helped We the People Michigan challenge the city of Detroit’s investment in acoustic gun detection, an unreliable AI-powered technology that threatened to exacerbate overpolicing of poor communities of color. Like bottom-up design, this partnership approach values communities as experts rather than simply consumers, nurturing a technological future that reflects public needs and democratic vision.
Limiting Inequity and Injustice through Regulation
Equitable design, driven by the needs of marginalized communities, will do much to promote beneficial adoption of AI and prevent harms associated with the technology. However, we also know that developers will pursue profits and the technical challenges that interest them, without much concern for equity. With this in mind, AI technologies must be subject to regulation, and this regulation should occur before technologies come to market. In particular, technologies that disproportionately harm marginalized communities should be prohibited. The Biden administration has already taken some steps in this direction with a 2023 executive order that, among other things, calls on federal agencies to issue guidance on the use of AI in law enforcement, hiring, housing, and health care and directs the Federal Trade Commission to specify that algorithmic discrimination in access to credit is illegal. But a systematic regulatory process could do more to disincentivize the creation of unjust and inequitable AI.
Regulation could be accomplished through impact assessments, inspired by the 1970 National Environmental Protection Act, which requires development projects to undergo an environmental assessment and demands more extensive review of higher-risk interventions. In particular, technology impact assessment should be focused on equity, which would involve auditing the datasets and algorithms underlying AI tools to determine whether the outputs might discriminate against or otherwise harm marginalized communities.
Such evaluation also must extend beyond technical components of AI, because even a well-functioning system designed to pursue a worthwhile goal like crime reduction can perpetuate structural inequalities. Thus an equity-focused impact assessment should consider the social context in which the technology will be used: regulation should attend to characteristics of AI users and ensure that they are adequately trained to mitigate bias.
Such sociotechnical evaluation will require the work of experts across disciplines, including science, law, humanities, and the social sciences. And regulation, like design, will be incomplete without participation from marginalized communities. The input of minoritized people in regulating AI is essential; that input should be solicited and valued, and participation should be voluntary and compensated. This is a means of building trust while alleviating structural inequities in AI and innovation generally.
The input of minoritized people in regulating AI is essential; that input should be solicited and valued, and participation should be voluntary and compensated.
What we propose is a serious commitment, but we know that thoughtful sociotechnical assessment is productive. Consider the response of New York officials to concerns about the use of facial recognition in K–12 schools. The state’s Office of Information Technology Services analyzed benefits and harms of this particular deployment of the technology, with an eye toward both technical accuracy and the likelihood that the technology would exacerbate bias. Drawing on evidence from legal cases and scientific and social scientific research, the office found that even accurate systems would violate civil rights. In response, the state legislature banned the use of facial recognition in schools.
Harnessing Benefits of AI through Intellectual and Moral Change
Ensuring that AI advances, rather than harms, progress toward social equity and justice entails intellectual and moral change, not just new rules. Educators and research funders must promote equitable design, so that developers want to work with marginalized communities to learn about their needs and together build technologies that provide meaningful benefit. With this in mind, engineers must be sensitized to systematic biases in datasets and algorithms but also to methodologies that promote community partnerships capable of correcting inequities resulting from discrimination. And policymakers must be prepared to think creatively, attuning regulations not only to technical characteristics of AI products but also to those products’ equity impacts in real-life scenarios.
Bottom-up knowledge and the humility to keep learning from those in need: these are tools for ensuring responsible AI but also for realizing the immense potential of this emerging technology. AI can exacerbate social problems, but it can also be used to solve them. Alongside their obligation to prevent harm, policymakers, research funders, tech and university leaders, and STEM professionals have an opportunity to foster equity through innovation. That is where the true promise of AI lies.