Novel Technologies and the Choices We Make: Historical Precedents for Managing Artificial Intelligence
Artificial intelligence needs ongoing and meaningful democratic oversight. Understanding the history of how the early nuclear weapons complex, novel biotechnology, and polygraph testing were managed can inform AI governance today.
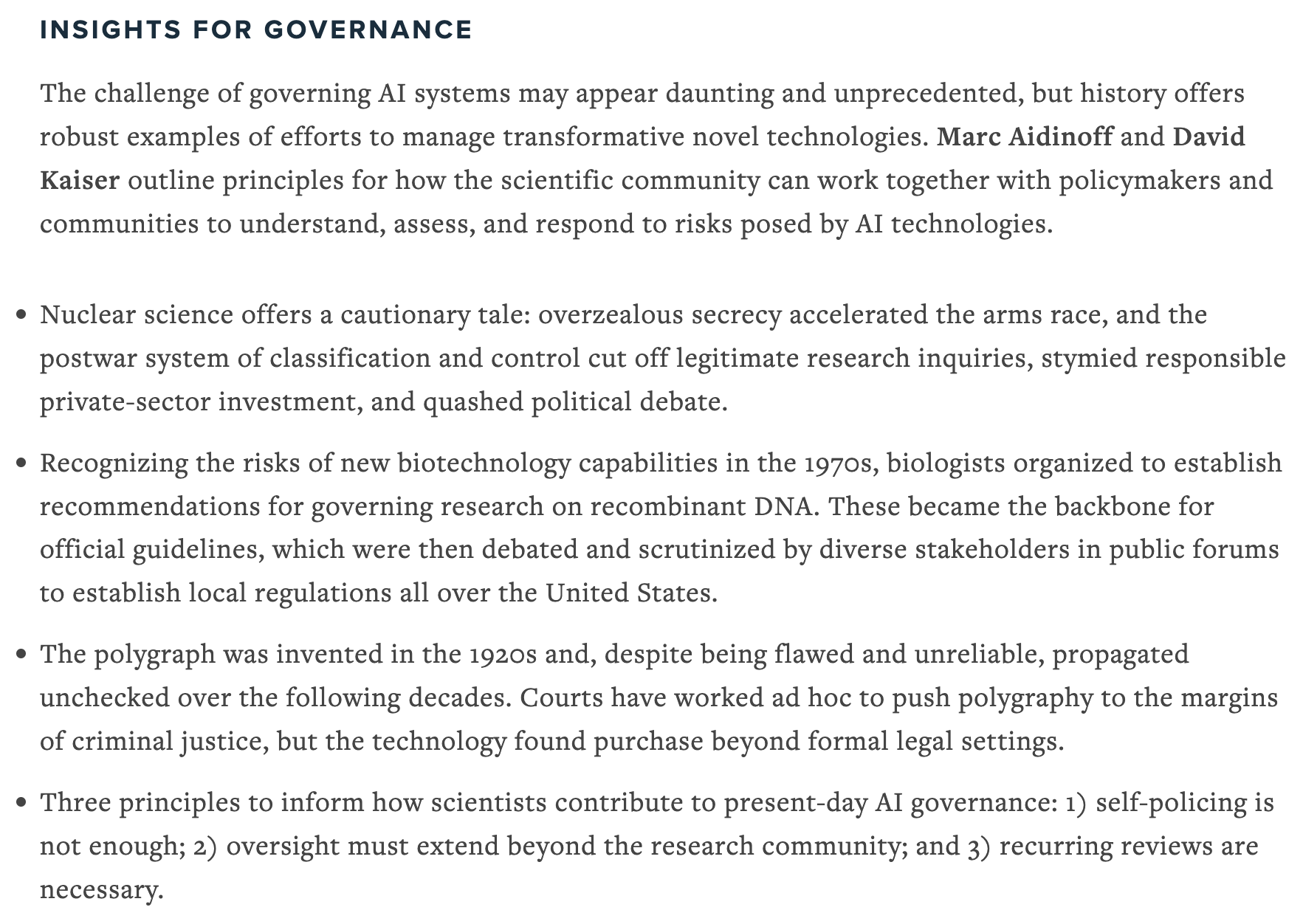
Scientific and technological innovations are made by people, and so they can be governed by people. Notwithstanding breathless popular descriptions of disempowered citizens cowed by technical complexity or bowing to the inevitable march of the new, history teaches that novel technologies like artificial intelligence can—indeed, must—be developed with ongoing and meaningful democratic oversight. Self-policing by technical experts is never enough to sustain an innovation ecosystem worthy of public trust. Contemporary AI might be a distinct technological phenomenon, but it too can be governed in the public interest.
History provides insights on how governance might proceed today. There is a robust empirical record of efforts to manage transformative technologies—a record of fits and starts, as wide-ranging constituencies work to make policies that advance the greater good. In this essay, we consider three examples: governance of the early nuclear weapons complex during the 1940s and 1950s, of novel biotechnology in the 1970s, and of polygraph testing and other forensic technologies that emerged over the last century.
In each instance, leaders of the scientific and technical communities sought to define and protect the public interest. Yet in none of these instances did scientists and technologists hold unilateral sway over how the new technologies would be assessed, deployed, or governed. The same is true for AI: technical experts will have their say, but their voices will be joined by others. And while no historical case offers a perfect analogy for present-day challenges, all three of these examples, understood side-by-side, help to identify realistic options for citizens and policymakers struggling to govern AI now.
Keeping Nuclear Secrets
Many commentators today compare the generative-AI rush with the dramatic efforts to build nuclear weapons during the Second World War, often calling for a “Manhattan Project” for AI. To some, the analogy with the Manhattan Project summons a coordinated, large-scale effort to surmount technical challenges. To others, it signals a need for careful control over the flow of information given the risks surrounding a high-stakes technology. Yet the history of nuclear secrecy reveals the limits of such a model for managing AI today.
There is a robust empirical record of efforts to manage transformative technologies—a record of fits and starts, as wide-ranging constituencies work to make policies that advance the greater good.
Research in nuclear science quickly became sensitive, as the path from basic discoveries to sprawling weapons programs was dizzyingly short. The first indication of nuclear fission came in December 1938; by April the following year, the German Reich Ministry of Education was banning uranium exports and holding a secret meeting on military applications of fission. That same month, the Japanese government launched a fission-weapons study, and several British physicists urged their government to jumpstart a weapons project by securing uranium ore from the Belgian Congo. In August 1939 US president Franklin Roosevelt received a letter drafted by émigré physicists Leo Szilard and Eugene Wigner and signed by Albert Einstein alerting the White House that nuclear weapons could exploit runaway fission chain reactions. A few weeks later, the Leningrad-based physicist Igor Kurchatov informed the Soviet government about fission’s possible military applications.
Amid worsening international relations, some scientists tried to control the flow of information about nuclear science. Beginning in spring 1939, Szilard urged a voluntary moratorium on publication of new findings in nuclear fission. When credit-hungry physicists refused, Szilard concocted a different plan: allow researchers to submit their articles to scientific journals—which would enable clear cataloging of discovery claims—but coordinate with journal editors to hold back certain papers until their release could be deemed safe. This scheme proved difficult to implement, but some journals did adopt Szilard’s recommendation. The physicists’ communication moratorium yielded some unexpected consequences: when Kurchatov and his Soviet colleagues noticed a distinct reduction in Physical Review papers regarding nuclear fission, they considered the grave potential of nuclear weapons confirmed and doubled down on efforts to convince Moscow that the matter must be taken seriously.
Szilard’s proposals focused on constraining access to information rather than regulating research itself. That distinction disappeared in June 1942, when the Allies’ patchwork of nuclear study groups were centralized under the auspices of the Manhattan Project. Officials exerted control over the circulation of information, materials, and personnel. The FBI and the Military Intelligence Division conducted background checks on researchers; commanding officer General Leslie Groves imposed strict compartmentalization rules to limit how much information any single individual knew about the project; and fissionable materials were produced at remote facilities in places like Oak Ridge, Tennessee, and Hanford, Washington.
After the war, secrecy routines were formalized with passage of the US Atomic Energy Act. Under the new law, whole categories of information about nuclear science and technology were “born secret”: classified by default and released only after careful review. The act also established a government monopoly on the development and circulation of fissionable materials, effectively foreclosing efforts by private companies to generate nuclear power. (Several of these provisions were amended in 1954 in order to foster private-sector efforts in nuclear power, with mixed results.)
Like Szilard in 1939, postwar scientists and engineers worked hard to shape the practices and norms of nuclear science and technology. But their illusions of control quickly collapsed amid Cold War pressures. For example, the newly established Federation of Atomic Scientists had some initial success lobbying lawmakers in favor of a civilian nuclear complex, but members soon became targets of a concerted campaign of intimidation. The FBI and the US House Committee on Un-American Activities pursued the federation, smearing several members with selective leaks and allegations of communist sympathies. Their attorneys were often denied access to information relevant to their cases under the pretext of protecting national security. The elaborate system of nuclear classification became a cudgel with which to silence critics.
Beyond its impact on individuals, the postwar nuclear-classification regime strained relationships with US allies—most notably Britain—even as it was ineffective in halting proliferation. Within a few years after the war, the Soviet Union built fission and fusion bombs of its own—efforts aided by wartime espionage that had pierced US military control. Arguably, overzealous secrecy accelerated the arms race.
Amid today’s calls to hold back the tide of new computational models and techniques, nuclear secrecy serves as a cautionary tale of bureaucratic overreach and political abuse. Undoubtedly there were good reasons to safeguard some nuclear secrets, but the postwar system of classification and control was so byzantine that legitimate research inquiries were cut off, responsible private-sector investment was stymied, and political debate was quashed. The academic community served as a weak but visible counterbalance, seeking to maintain the openness necessary for scientific progress and democratic oversight.
Controlling Biotechnology
Szilard’s first impulse was to persuade fellow scientists to stop publishing their most potent findings. In the mid-1970s, molecular biologists went further. Led by Stanford’s Paul Berg and colleagues at other elite universities and laboratories, scientists pressed for a halt not only to publication but also to research in the new area of recombinant DNA (rDNA). Their efforts included the famous Asilomar meeting of February 1975, which is routinely cited to this day as the preeminent example of scientists successfully and responsibly governing risky research. Yet, much like Szilard’s calls for nuclear scientists to self-censor, biologists’ self-policing was actually a small part of a much larger process. Responsible governance was achieved, but only after careful, protracted negotiation with stakeholders well beyond the scientific community.
Amid today’s calls to hold back the tide of new computational models and techniques, nuclear secrecy serves as a cautionary tale of bureaucratic overreach and political abuse.
Berg and his fellow biologists appreciated the potential benefits of rDNA techniques, which allowed scientists to combine fragments of genetic material from multiple contributors to create DNA sequences that did not exist in any of the original sources. But the group also foresaw risks. Pathogenic bacteria might acquire antibiotic-resistant genes, or carcinogenic genes might be transferred to otherwise harmless microorganisms. And if the Manhattan Project was carried out in remote, top-secret sites, rDNA experimentation involved benchtop apparatus found in nondescript laboratories in urban centers. What would protect researchers and their neighbors from leaks of dangerous biological materials? As Massachusetts Institute of Technology (MIT) biologist David Baltimore recalled after meeting Berg and others to brainstorm, “We sat around for the day and said, ‘How bad does the situation look?’ And the answer that most of us came up with was that …, for certain kinds of limited experiments using this technology, we didn’t want to see them done at all.” Berg, Baltimore, and the rest of their group published an open letter calling for a voluntary moratorium on rDNA research until risks were assessed and addressed. The request was met with considerable buy-in.
By the time their letter appeared in Science, Nature, and the Proceedings of the National Academy of Sciences, the Berg group had been deputized by the National Academy to develop recommendations for the National Institutes of Health (NIH). They convened again, this time with more concerned colleagues, in February 1975 at the Asilomar Conference Grounds in Pacific Grove, California. The Asilomar group, consisting almost entirely of researchers in the life sciences, recommended extending the voluntary research moratorium and proposed a framework for assessing risks and establishing containment facilities for rDNA experiments. In June 1976 the Asilomar recommendations became the backbone of official guidelines governing rDNA studies conducted by NIH-funded researchers.
On the very evening in June 1976 when the NIH guidelines were announced, the mayor of Cambridge, Massachusetts—home to famously difficult-to-govern research institutions like Harvard University and MIT—convened a special hearing on rDNA experimentation. “No one person or group has a monopoly on the interests at stake,” Mayor Alfred Vellucci announced. “Whether this research takes place here or elsewhere, whether it produces good or evil, all of us stand to be affected by the outcome. As such, the debate must take place in the public forum with you, the public, taking a major role.” And so began a months-long effort by area scientists, physicians, officials, and other concerned citizens to devise a regulatory framework that would govern rDNA research within city limits—under threat of a complete ban if the new Cambridge Experimentation Review Board failed to agree on rules that could pass muster with the city council.
Much like Szilard’s calls for nuclear scientists to self-censor, biologists’ self-policing was actually a small part of a much larger process. Responsible governance was achieved, but only after careful, protracted negotiation with stakeholders well beyond the scientific community.
The local board held public meetings twice weekly throughout autumn 1976. During the sessions, Harvard and MIT scientists had opportunities to explain details of their proposed research to nonspecialists. The board also hosted public debates over competing proposals for safety protocols. Similar civic groups hashed out local regulations in Ann Arbor, Michigan; Bloomington, Indiana; Madison, Wisconsin; Princeton, New Jersey; and Berkeley and San Diego, California. In none of these jurisdictions did citizens simply adopt the Asilomar/NIH guidelines. Rather, there was thorough scrutiny and debate. Cambridge residents, for example, called for the formation of a biohazards committee along with regular inspections of rDNA labs, exceeding federal requirements. Only after the board’s extensive, sometimes thorny negotiations did the city council vote to adopt the Ordinance for the Use of Recombinant DNA Molecule Technology within Cambridge. This was February 1977, two years after the Asilomar meeting.
With the ordinance in place, Cambridge quickly became a biotechnology juggernaut, earning the nickname Genetown. City officials, university administrators, laboratory scientists, and neighbors had worked together to construct a regulatory scheme within which innovative scientific research could thrive, both at universities and at spin-off companies that soon emerged. Public participation took time and was far from easy, but it proved essential for building trust while avoiding Manhattan Project–style monopolies.
Unregulated Forensic Science
Whereas Szilard and Berg tried to craft guardrails around the scientific work they were developing, in 1921 physiology student and police officer John Larson was eager to deploy his latest innovation: the cardio-pneumo-psychograph device, or polygraph.
The result, over the course of decades, has been the unchecked propagation of an unreliable technology. Courts, with input from scientific experts, have worked in their ad hoc way to push polygraphy to the margins of criminal justice. But the polygraph has not been subject to the sorts of democratic oversight and control that helped to ensure the safety and utility of rDNA research. Courts might similarly clamp down on algorithmic facial recognition, the AI-driven forensic technology of the moment; but facial recognition, too, is already commonplace and unregulated. Indeed, public narratives about seemingly miraculous yet flawed technologies can aid in their escape from oversight, creating havoc. There is a lesson here in the importance of both public intervention by concerned scientists—before risky technologies become commonplace—and the need for continuing regulatory scrutiny.
Havoc was not Larson’s goal. Like many early twentieth-century intellectuals, he was convinced that measurements of the body could surface what was buried in the mind. The nineteenth-century physician Étienne-Jules Marey took physical measurements to reveal stress, in hopes that these would in turn reveal interior truths. And by 1917, psychologists and married couple William Moulton Marston and Elizabeth Holloway Marston invented a form of the polygraph. Within a few years, as historian Ken Alder has carefully documented, Larson made two crucial upgrades to the Marstons’ approach. First, Larson’s machine took continuous blood pressure measurements and recorded them as a running line, so that a polygraph operator could monitor changes relative to a baseline. Second, Larson partnered with law enforcement.
The polygraph has not been subject to the sorts of democratic oversight and control that helped to ensure the safety and utility of rDNA research.
In the spring of 1921, Larson tried out his technology to solve a real crime, a potboiler drama involving a missing diamond presumed stolen by one of 90 women living in a boardinghouse. The thief, whose recorded blood pressure did drop precipitously during her interrogation, eventually confessed after days of additional questioning. Eager for gripping narratives, journalists gravitated to the cardio-pneumo-psychograph—except, to Larson’s chagrin, the press renamed his device the “lie detector.” And some law enforcement figures were as enthusiastic as the reporters covering their police departments. August Vollmer, chief of police in Berkeley, California, was an early adopter of the cardio-pneumo-psychograph, believing that the technology could help his department overcome its poor reputation. The public viewed police as corrupt and overly reliant on hunches and personal relationships; Vollmer thought Larson’s methods, though unproven, might lend policework the patina of scientific expertise, bolstering support.
As the press attention suggests, the polygraph was a charismatic technology. Having captured the public interest, the so-called lie detector found ready purchase beyond formal legal settings. Some uses were benign—for instance, market researchers turned to polygraphs in hopes of understanding what drew audiences to particular films or actors. But the stakes of deploying this unreliable technology grew in other domains, as when employers turned to the polygraph to screen for job suitability.
Judges were less willing to accept the polygraph, which became clear during the 1922 trial of one James Frye. Frye had confessed to murder but later claimed that his statement had been coerced. A polygraph test validated Frye’s claim, but a judge rejected the result, claiming it could not serve as evidence. This led to the so-called Frye test, in which a federal court held that scientific evidence was inadmissible unless it was derived from methods enjoying “general acceptability” within the scientific community. This judicial test, which the polygraph failed, reflected a belief that juries would be swayed by supposedly objective scientific evidence. Such evidence, then, had to be held to a high standard.
For its part, the scientific community repeatedly mobilized to limit the use of polygraphs in court. As the US Office of Technology Assessment (OTA) concluded in a 1983 report, there was “limited scientific evidence for establishing the validity of polygraph testing.” But resistance to polygraph testing in the criminal justice sphere was matched by exuberance elsewhere. The same OTA report estimated that, outside of the federal government, more than a million polygraph tests were administered annually within the United States for hiring purposes. In 2003 the National Academies led another effort to scrutinize the reliability of the polygraph. The resulting report has played a crucial role in keeping polygraphs out of courtrooms.
In contrast to polygraphy, other science-based techniques such as fingerprint analysis have a more secure place in US legal proceedings. Fingerprint-based identification is far from perfect, but it has for decades been subject to standardization and oversight, and expert witnesses must be trained in the technique. Moreover, high-profile mistakes have catalyzed meaningful ameliorative review. Expert panels have responded to errors by reassessing the scientific bases for fingerprint identifications, updating best practices for their use, and developing new methods for training practitioners.
Algorithmic facial recognition has followed a trajectory more like that of the polygraph than of fingerprinting. Despite its significant and well-documented flaws, facial recognition technology has become ubiquitous in high-stakes contexts outside the courtroom. The US National Institute of Standards and Technology (NIST) recently evaluated nearly 200 facial recognition algorithms and found that almost all demonstrated enormous disparities along demographic lines, with false positives arising a hundred times more often when the technologies were applied to images of Black men from West Africa as compared to images of white men from Eastern Europe. The NIST tests also found systematically elevated rates of false positives when the algorithms were applied to images of women across all geographical regions as compared to men. Given such clear-cut biases, some scholars have called for more inclusive datasets, which in theory could broaden the types of faces that can be recognized. Other commentators have argued that inclusion would simply put more people at risk.
Many research papers have focused on ways to mitigate biases in facial recognition under pristine laboratory conditions, but uncorrected commercially available algorithms are already having substantial impact outside the walls of research facilities. In the United States, law enforcement jurisdictions can and do purchase commercial facial recognition technologies, which are not subject to regulation, standardization, or oversight. This free-for-all has led to multiple reports of Black men being wrongfully arrested. These real-world failures, which exacerbate long-standing inequities in policing, are likely to worsen in the absence of oversight. There exist today more than a billion surveillance cameras across 50 countries, and within the United States alone, facial images of half the adult population are already included in databases accessible to law enforcement.
NIST recently evaluated nearly 200 facial recognition algorithms and found that almost all demonstrated enormous disparities along demographic lines, with false positives arising a hundred times more often when the technologies were applied to images of Black men from West Africa as compared to images of white men from Eastern Europe.
Like the polygraph, facial recognition technologies have created a certain amount of chaos beyond law enforcement settings. There have been sensational claims that far outstrip technical feasibility—for instance, that algorithmic analysis of facial images can determine an individual’s sexual orientation. Meanwhile, private vendors are scooping up as many facial images as they can, almost always from platforms whose users have not granted permission for, and are unaware of, third-party data collection. In turn, facial surveillance is now deployed in all sorts of contexts, including schools, where the technology is used to monitor students’ behavior. Facial recognition is also being used to prevent access to venues and even for job screening.
Three Principles for Researchers in AI Governance
AI policy is marked by a recurring problem: a sense that AI itself is difficult or even impossible to fully understand. Indeed, scholars have shown how machine learning relies on several forms of opacity, including corporate secrecy, technical complexity, and unexplainable processes. Scientists have a special obligation to push against claims and realities of opacity—to demonstrate how the consequences of complex technologies can be explainable and governable. As our historical examples show, at its best the scientific community has worked to assemble coalitions of researchers and nonresearchers to understand, assess, and respond to risks of novel technologies. History offers reason to hope that building such collective processes around AI is possible, but also reasons to worry that such necessary work will be hard to sustain.
Three principles are apparent across these historical examples—principles that should inform how scientists contribute to present-day AI governance. First, self-policing is not enough. Researchers’ voluntary moratoria have rarely, if ever, proven sufficient, especially once high-impact technologies escape controlled laboratory settings. Scientists and engineers—though eager to act justly by putting bounds around novel technologies and mitigating risks—have never been good at anticipating the social and political lives of their innovations. Researchers did not predict the rise of an elaborate Cold War secrecy infrastructure, the robust public debate surrounding rDNA experiments, or popular enthusiasm for the polygraph. Because accurate predictions concerning real-life responses to novel technologies are beyond the scope of scientific expertise, scientists and engineers cannot be expected to know where exactly the boundaries around novel technologies should lie.
At its best the scientific community has worked to assemble coalitions of researchers and nonresearchers to understand, assess, and respond to risks of novel technologies.
Second, oversight must extend beyond the research community. Broad input and regulatory supervision have repeatedly proved necessary to sustain innovation ecosystems. Extended debate and negotiation among researchers and nonspecialists can build public trust and establish clear regulatory frameworks, within which research can extend across academic and private-sector spaces.
Finally, recurring reviews are necessary. Specialists and nonexpert stakeholders should regularly scrutinize both evolving technologies and the shifting social practices within which they are embedded. Only then can best practices be identified and refined. Such reviews are most effective when they build upon existing civic infrastructures and expectations of civil rights. Civic organizations with long histories of advancing rights and liberties must have an empowered role in review processes.
Today’s AI technologies, like many predecessors, are both exciting and fraught with perils. In the past, when scientists and technologists have spoken decisively about risks, articulated gaps in knowledge, and identified faulty claims, they have often found collaborators beyond their research communities—including partners in government, the legal system, and the broader public. Together, these communities at times have successfully established governance frameworks within which new technologies have been developed, evaluated, and improved. The same commitment to genuine partnerships should guide governance of AI technologies. Any other approach would put at risk the enormous potential of AI as well as the societies that stand to gain from it.