A Human Rights Framework for AI Research Worthy of Public Trust
Artificial intelligence researchers regularly conduct social experiments relying on data from participants who haven’t agreed to take part. To earn public trust, researchers need to reorient computational research toward respect for human rights by adopting a robust AI ethics protocol.
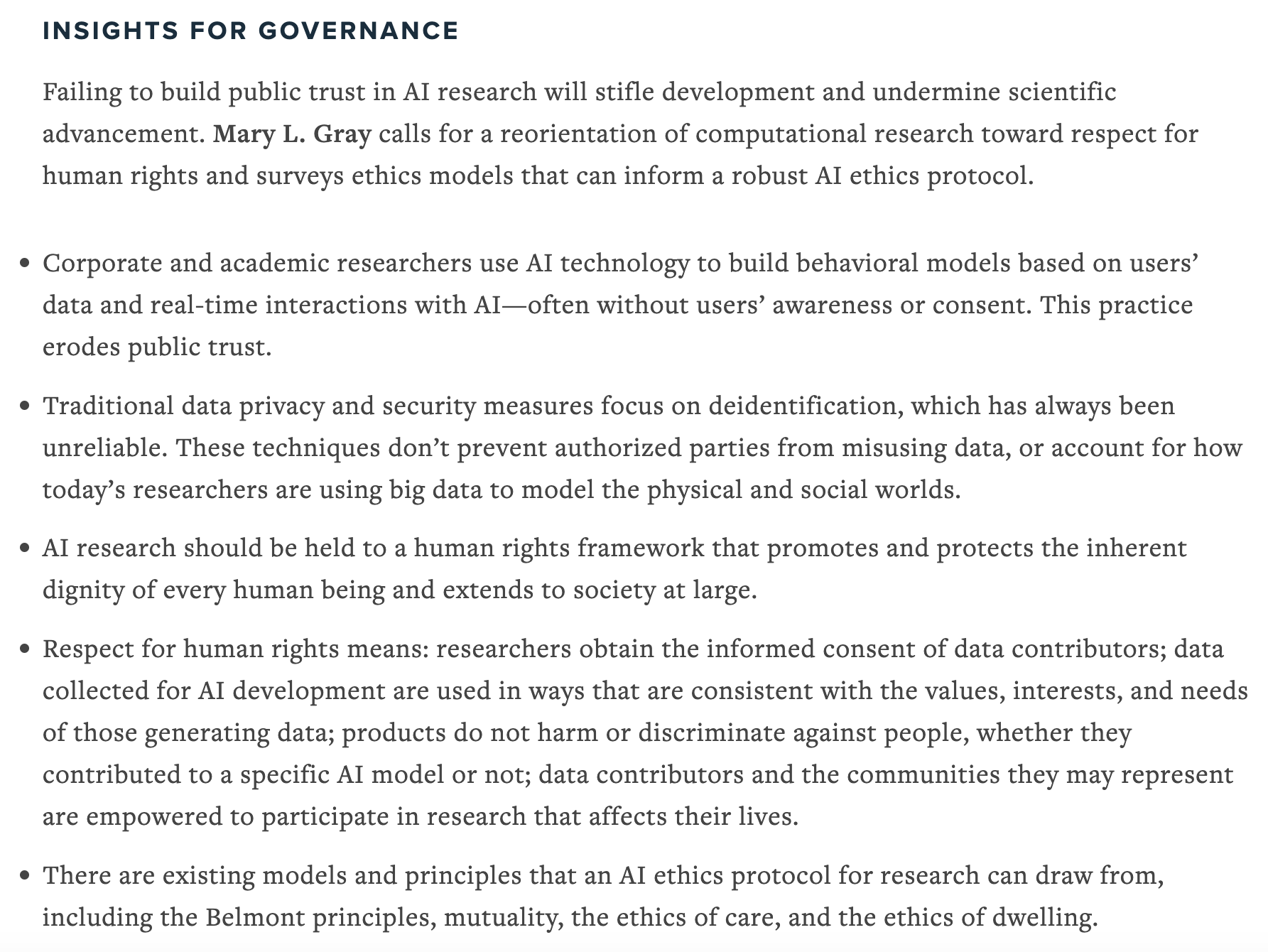
In 2014, researchers at Cornell University and Facebook joined forces for an experiment. They wanted to find out whether emotional contagion occurs on social media—whether the expressions of emotion showing up in our newsfeeds influence the way we ourselves feel. The study results were clear and important, confirming that emotional contagion is common on social media, as it is in in-person interaction. But while scientists celebrated a significant finding, the public became incensed. The project involved manipulating the feeds of nearly 700,000 Facebook users and studying their responses without their knowledge or informed consent, leading to widespread accusations of ethical lapses.
A decade later, the commercial launch of generative AI has provoked similar uproar. After all, many of the most popular and useful AI tools involve social experiments relying on participants who haven’t agreed to take part. Researchers in corporate and academic settings use AIs to build statistical models of human behavior based on user data and on users’ real-time interactions with AIs. Yet users are seldom consulted about their willingness to be analyzed by machine-learning algorithms or to be manipulated in the process. Whether or not you use AI tools, you have probably been “opted in” to an experiment on people. And if you are using an AI tool, you may be a sort of lab rat yourself.
When the hidden experiments of scientists come into view, the public tends to see them as beyond the bounds of decency—and possibly illegal. AI-based research, in other words, has a public trust problem, with potentially grave consequences. A collapse in trust would not only stifle AI development but also undermine the huge range of research projects that might use AI to advance scientific inquiry.
If AI is to fulfill its promise, the researchers who develop and take advantage of it must build and maintain public trust. And the more that scientific and technological innovation depends on learning from people’s data and decisionmaking, the more trust researchers will need to call on. Yet the path they are currently taking risks failure. How can AI researchers earn public trust?
Researchers in corporate and academic settings use AIs to build statistical models of human behavior based on user data and on users’ real-time interactions with AIs. Yet users are seldom consulted about their willingness to be analyzed by machine-learning algorithms or to be manipulated in the process.
Achieving an AI research paradigm deserving, and nourishing, of public trust will require reforms. For one thing, the deidentification techniques intended to protect the privacy and security of those contributing data to research projects should be fundamentally overhauled. These techniques have never been reliable, and it is past time that corporate and academic researchers relying on information scraped from the internet commit to a higher standard of data stewardship. But that alone will not be enough. Scientists must be, more generally, committed to respect for the human rights of individuals and groups wittingly and unwittingly participating in their experiments.
A key means of reorienting computational research toward respect for human rights is the adoption of a robust AI ethics protocol. Existing protocols, such as the Belmont principles for human subjects of research, are not sufficient unto themselves. But they can be updated and expanded for the AI age, in hopes of establishing a relationship of care and trust between researchers and society at large.
Moving Beyond Failed Data Privacy and Security Measures
Remember AOL? In 2006 the company released a snapshot of users’ search data: 20 million queries from over 650,000 users were collected in a single text file and posted to a publicly accessible webpage. The file contained no user names or email addresses but did include numerical identifiers for each user and their queries. The assumption was that this would be sufficient to protect users’ identities while still providing the research community a bounty of data from which to learn. But it took two New York Times reporters only a few days to crack the case. Soon they were interviewing a Georgia woman whom they identified on the basis of her queries, which obliquely revealed details about who she might be.
Nearly 20 years later, little progress has been made toward strengthening deidentification methods, even as computer scientists have long understood how brittle they are. And even less progress has been made toward a more expansive ethical vision for computing research.
Security and privacy are important aspects of data protection, but they cannot address the ethical and social implications of, say, training an AI to model group behaviors based on health and social data. Security and privacy measures also do not prevent the misuse of data by authorized parties. For example, a retailer could use data to which they are legally entitled to find out information about customers that they do not wish to reveal, such their health status or whether they are pregnant. There need be no breach of data security in such cases, which is another way of saying that protections do not ensure respect for the preferences and expectations of people who contribute their data.
Yet many researchers continue to treat data security—with the limited goal of deidentification—as their principal and perhaps only ethical obligation. This narrow approach was arguably defensible when computing research did not engage deeply in people’s everyday lives. But today’s scientists, spanning scholarly and corporate domains, use big data to model the physical and social worlds, especially human decisionmaking. Under these circumstances, old-style data security is even less effective than it used to be.
A Human Rights Approach: Taking Trust and Participation Seriously
Researchers’ norms around security and privacy are clearly inadequate—both to meet society’s expectations and to protect people from AI-driven mishaps. Computing-dependent research must be subject, then, to a higher standard, which I submit is the standard of human rights. Researchers must respect the human rights of individuals who contribute to AI models and of those groups presumed to have been modeled. That is to say, respect for human rights must extend not just to research participants but to society at large. Public confidence, and with it the future of AI development and AI-driven science, hinges on the adoption of this high standard.
Security and privacy are important aspects of data protection, but they cannot address the ethical and social implications of, say, training an AI to model group behaviors based on health and social data. Security and privacy measures also do not prevent the misuse of data by authorized parties.
Respect for human rights in research means more than providing for data security. This has been a consensus view since at least 1964, when the World Medical Association adopted the Declaration of Helsinki, recognizing the right of individuals’ to decide whether or not to participate in medical experiments. Under a human rights framework, researchers actively promote and protect the inherent dignity of every human being by taking responsibility for securing their fundamental rights and freedoms. Respect for human rights requires, for instance, that the health and other social data collected for AI development are used in ways that are consistent with the values, interests, and needs of those generating data—and it requires that the resulting products not harm or discriminate against people, whether they contributed to a specific AI model or not. Respect for human rights also entails that data contributors and the communities they may represent are empowered to participate in research that affects their lives.
Participation necessitates, at the very least, informed consent. It can also involve deeper forms of exchange between researchers and data contributors, such as consultation, co-creation of research projects, and co-ownership of research outputs. Participation can enhance trust in and acceptance of AI by fostering transparency and therefore accountability. And broad participation, cutting across socioeconomic and demographic groups, can enhance the quality and relevance of AI models by ensuring that the social data on which they are based reflect the diversity and complexity of human experience.
Only when the effects of AI models reflect the interests of the communities on which they are based will we know that research is truly in line with the obligation to respect human rights. If this seems like an impossibly high bar to clear, that only goes to show how little care is given to data contributors and the groups they are said to represent right now.
Researchers must respect the human rights of individuals who contribute to AI models and of those groups presumed to have been modeled.
The award-winning ASL Citizen study exemplifies the sort of human rights–centered science I have in mind: it is a rare case of computing research that both respects community norms surrounding data collection and model-building and produces results that the data-contributing community values. This study aims to address the relative absence of native signers from American Sign Language (ASL) datasets used by machine learning models. The ASL Citizen study created and released the first machine-readable sign language dataset drawn from native signers, containing about 84,000 videos of 2,700 distinct signs from ASL. This dataset could be used for ASL dictionary retrieval and to improve the accessibility of what are otherwise voice-activated technologies.
The ASL Citizen study respects the autonomy and dignity of contributing signers by obtaining their informed consent, ensuring their privacy and security, and giving them the option to withdraw their data at any time. The study also respects the diversity and complexity of the US signing community by involving researchers and collaborators who sign and by collecting data from signers with varied backgrounds, experiences, and signing styles. Further, the study is clearly beneficial: it creates a large and diverse dataset that can advance the state of the art in sign language recognition, enabling new technologies that can improve communication among signers and make useful tools available to them. Meanwhile, harms are minimized by ensuring the quality and validity of data and models through the use of rigorous methods for data collection, annotation, evaluation, and dissemination. By releasing their dataset and code under open licenses, and by providing detailed documentation of data and models, the study authors invite scrutiny and accountability. Finally, the study promotes fairness and equity by addressing the needs and interests of a community underrepresented in computing research to date.
The contrast between the ASL Citizen study and the 2014 Facebook-Cornell emotional contagion study could hardly be starker. Both of these studies produced valuable results, but the emotional contagion project, by failing to respect people’s fundamental rights to determine their role in scientific studies, did so at the cost of undercutting trust in the scientific community and in computing research.
Principles and Tools for Ethical AI-Driven Research
A good starting point for an ethics of AI research—guidance that, if followed, would promote public trust—might be the Belmont principles. Produced by a federally chartered panel of experts over the second half of the 1970s, the Belmont principles are the philosophical foundations of human-subjects research ethics in biomedicine and behavioral health in the United States. But the principles could also apply to a wider range of research involving people contributing data to AI models.
Only when the effects of AI models reflect the interests of the communities on which they are based will we know that research is truly in line with the obligation to respect human rights.
The Belmont framework includes three core principles: respect for persons, beneficence, and justice. First, respect for persons requires that human subjects are treated as autonomous agents who can freely consent or decline to participate in research. In addition, vulnerable people and those who experience diminished autonomy—such as children, incarcerated people, and people with certain cognitive challenges—are to be protected from coercion and exploitation. Second, beneficence requires that human subjects are not exposed to unnecessary or excessive harms or risks and that the potential benefits of research outweigh the potential harms or risks. Finally, justice requires that human subjects are selected fairly and equitably, and that the benefits and burdens of research similarly are distributed fairly and equitably across society.
Computing research could be significantly improved on the basis of these guidelines, but they cannot be the whole of AI ethics. It has been widely noted that the Belmont principles, which were designed to guide ethical interaction between discrete researchers and research participants, are too individualistic to address social life. This criticism is well taken and is certainly applicable to AI research, given its focus on modeling human decisionmaking at scale. Even rigid conformity to Belmont principles may not ensure the interests of groups said to be represented by AI models.
That being the case, we might look to complementary perspectives from humanist scholars whose work implicates research ethics. These ideas might enrich our sense of what constitutes ethical research in the public interest, motivating investigators—in particular, those using AI—to respect human rights in society broadly and carry out their work in a manner that leaves the public confident in the probity of scientists.
One enriching perspective comes from the concept of mutuality, which emphasizes the interdependence and reciprocity of human beings and the moral significance of caring for others as well as ourselves. Mutuality challenges individualistic assumptions of the liberal tradition and proposes a more relational approach to moral reasoning and action. Whereas liberal ethics emphasizes each person’s possession and vindication of rights, mutuality emphasizes negotiation across barriers of difference and disagreement. With this in mind, a researcher dedicated to mutuality might convene their project’s multiple stakeholders, who will determine together what exactly are the risks and rewards of the research and how these will be distributed. A commitment to mutuality has the potential to foster an inclusive and democratic AI research culture, where the voices and interests of diverse data contributors and their communities are heard and respected.
Scientists could also embrace a more useful and trustworthy research paradigm through a commitment to the ethics of care, a feminist theory associated above all with the philosopher Joan Tronto. Tronto defines care as “a [human] activity that includes everything we do to maintain, continue, and repair our ‘world’ so that we can live in it as well as possible.” We care in all sorts of ways—we care about, take care, give care, and receive care from others. All of these involve ethical responsibilities that Tronto describes and that are worthy of careful consideration. As the feminist anthropologist Ramona Perez has argued, researchers might turn to care ethics to orient themselves to their own motivations and obligations and to the effects of their work on both research participants and society at large.
Finally, researchers might invest in the ethics of dwelling. The social theorist Jarrett Zigon contrasts dwelling—a mode of being-in-the-world that is attuned to the ethical demands and possibilities of one’s situation—with acting, a mode of being-in-the-world that is guided by norms and rules. Zigon argues that dwelling is in fact the primary mode of ethical life, as people respond creatively to circumstances as they encounter them, seeking to transform themselves and their worlds accordingly. As a framework for scientific inquiry, dwelling involves building open and ongoing relationships between researchers and research participants, with scientists learning how the contexts of participants’ daily lives affect their behavior and their needs. In the AI research field, strong relationships with data contributors will help scientists understand what they are modeling so that they can develop more sophisticated systems that reflect the complexity of human experience. Imagine both the ethical and scientific benefits of building on rather than ignoring the diversity and unpredictability of human life!
The Belmont principles, which were designed to guide ethical interaction between discrete researchers and research participants, are too individualistic to address social life.
These may seem like abstract ideas, but fortunately there are also concrete projects that researchers can look to for tools and inspiration—projects that foster participatory and human rights–respecting approaches to AI model-building. Investigators working with people and their data should take note of Data for Black Lives, which mobilizes scientists, activists, and organizers to use data in ways that foster beneficial change for Black people. The Our Data Bodies project traces effects of AI models on marginalized peoples’ opportunities, such as their ability to obtain decent housing and public assistance. The AI Blindspot framework offers a method for identifying and mitigating AI-based discrimination by engaging diverse stakeholders and perspectives. And the Data Nutrition Project promotes transparency in AI model-building by evaluating the completeness, accuracy, and representativeness of datasets and assembling the findings in an easy-to-digest label somewhat like those found on food packaging.
Importantly, these projects are informed by the participation of subject matter experts outside the data-science community, including data contributors, social scientists, and humanists. To rethink their narrow attention to security and privacy and instead consider human rights broadly, scientists must be willing to learn from the rest of society. Indeed, they should be more than willing—they should be eager to embrace the opportunity. Collaboration builds trust and, in AI research, improves outcomes by helping scientists better understand the real-world effects their models might have.
A Future Built on Trust
From where I sit, with one foot in academia and the other at an industry-based computing research center, it is clear that AI governance is a near-term problem. It needs to be addressed now; we can’t wait to evaluate what happens after more AI models have been built and deployed. By then, public trust in computing research may have run out, and opportunities to do real good with AI studies could be squandered.
We need governance on the basis of sound ethical principles now because we need to be building public trust—now. This is the resource that will underlie the robustness of AI systems, especially in sensitive domains such as health, education, and security. Scientific communities should therefore be working overtime to build public confidence in AI. Measures of public trust in our practices—how we engage study participants, communicate the value of our study questions, and steward contributors’ data—might serve as some of our best benchmarks of research success. Then too, the public will be focused on outcomes: scientists should prioritize the development of AI models that, beyond simply incorporating bias mitigation, support the distribution of benefits to the least advantaged.
In other words, both the process and effects of research should be governed through an ethical framework designed to secure human rights—those of research participants and of society at large. Belmont-style principles can help with the process side, framing researchers’ obligations to data contributors. And other perspectives help us understand how to realize good outcomes at social scale, especially among the groups modeled by AI. On the basis of this richer ethical framework, researchers can preserve their ability to do science using advanced computational techniques, generating both valuable knowledge and the public trust that is the basis of their best work.