A Justice-Led Approach to AI Innovation
To ensure that innovation enhances freedom and promotes equality, research and development should be governed by an ethical framework grounded in a conception of justice fit for a pluralistic, open society.
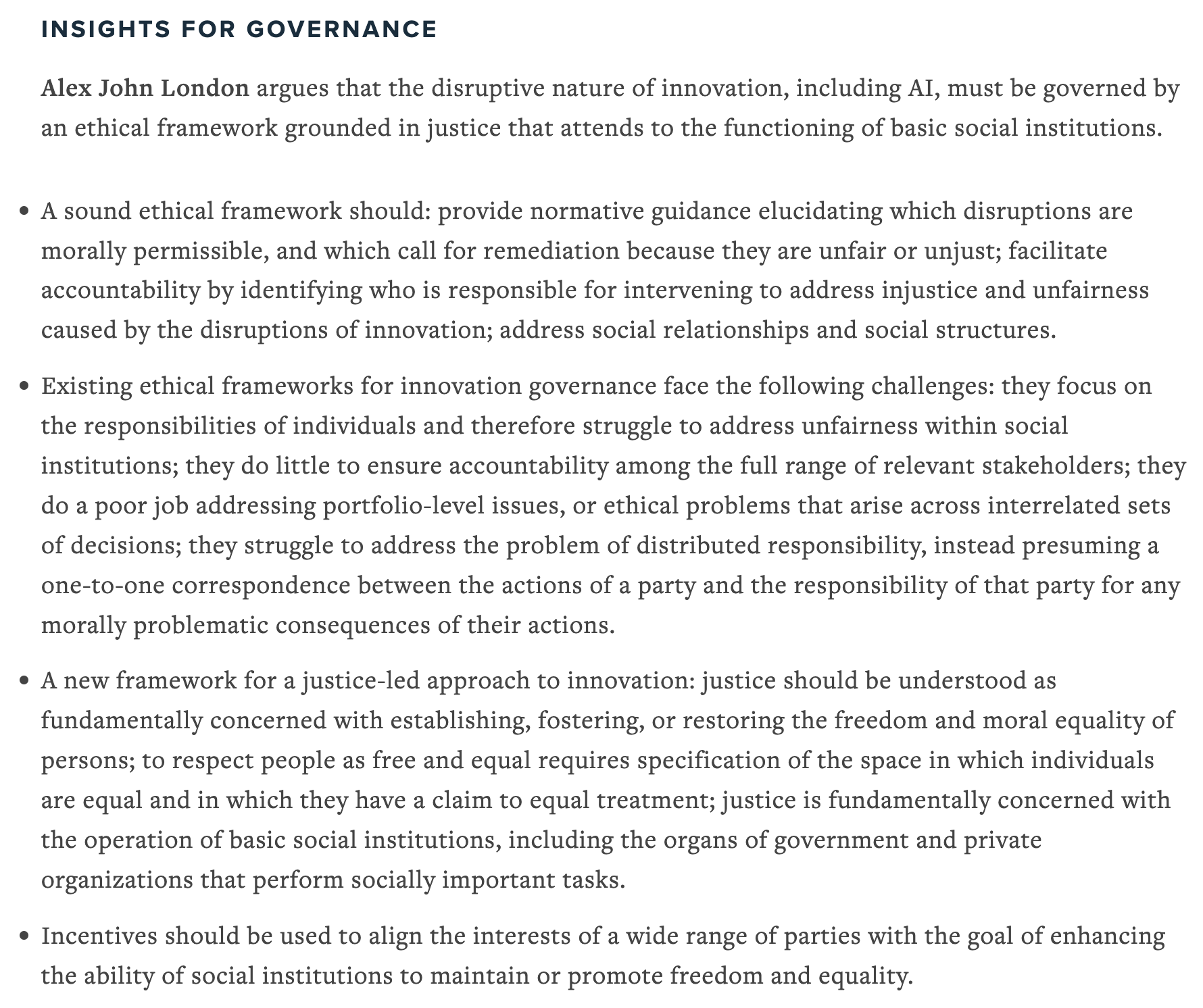
“As it is useful that while mankind are imperfect there should be different opinions, so it is that there should be different experiments of living; that free scope should be given to the varieties of character, short of injury to others; and that the worth of different modes of life should be proved practically, when any one thinks fit to try them.”
—John Stuart Mill, On Liberty
Innovation is disruptive; it changes either the ends we can achieve or the means of achieving established ends. This is certainly true of innovation in artificial intelligence. AI has been used, for example, to increase the capacity of persons with disabilities to access and share information—but has also enabled novel forms of deception, so that now one can create realistic photos, audio, and video of political figures doing or saying whatever one wishes.
To ensure that innovation enhances freedom and promotes equality, research and development should be governed by a sound ethical framework. Such a framework should fulfill at least the following three criteria. First, it should provide normative guidance elucidating which disruptions are morally permissible, and which call for remediation because they are unfair or unjust. Second, the framework should facilitate accountability by identifying who is responsible for intervening to address injustice and unfairness caused by the disruptions of innovation.
Third, the innovation-governance framework should address social relationships and social structures: it should consider how innovation influences divisions of labor and distributions of goods and harms across society over time, not only with respect to the immediate conduct of individuals. Current frameworks for applied ethics fall short in this regard because they focus on first-order interactions—the direct effects of discrete interactions between specific parties, such as scientific investigators and participants in research studies. Responsible governance of AI innovation, however, will have to address not just first-order interactions but also higher-order effects of portfolios of transactions among wide-ranging parties, such as effects of algorithmic policing tools on oppressed communities.
This entails that the framework for responsible governance be grounded in something more than ethics guidance. Instead, it must be grounded in a conception of justice fit for a pluralistic, open society. Exactly what constitutes justice is difficult to describe in brief, but we can at least get at the basics here. A just society, crucially, is not one in which all people agree on what constitutes a morally good life; such societies cannot exist, and efforts to create them are necessarily oppressive. Rather, a just society is one in which all people are equally free to pursue their own ideas about what a good life might be. To achieve justice, then, we need social institutions that promote the freedom and moral equality of all people. And so, to the extent that innovations in AI and other technologies might threaten some people’s freedom or their standing as moral equals, we need institutions capable of correcting these wrongs and promoting social arrangements that better secure people’s freedom in the face of technological change.
Existing Ethical Frameworks Neglect Justice
Perhaps the most influential approach to responsible regulation of innovation in the United States is that of the Belmont Report, published in 1979 by the US National Commission for the Protection of Human Subjects of Biomedical and Behavioral Research in response to revelations of abuse in biomedical and behavioral research. The report articulates principles of nonmaleficence, beneficence, respect for autonomy, and justice, and it provides guidance on how these principles should regulate interactions between scientific investigators and the humans who participate in their research projects. These principles and guidelines underlie the specific regulatory requirements governing institutional review boards, which oversee federally funded investigations in biomedicine and behavioral health.
The framework for responsible governance be grounded in something more than ethics guidance. Instead, it must be grounded in a conception of justice fit for a pluralistic, open society.
Although the Belmont approach has never been perfect in implementation, the ethics framework it created provides credible assurance that regulated research studies will respect study participants’ rights and interests. Given the esteem the Belmont system has earned, it should be no surprise that concerned parties increasingly argue for its extension to AI innovation. While the Belmont principles were designed to govern innovation in biological and behavioral sciences, many proposed AI-ethics frameworks now promote Belmont-style governance.
Yet the Belmont principles are insufficient to govern AI innovation because they do not specify the requirements of justice in a way that captures the role of social institutions and distributions of social labor in shaping innovation and its impacts. Rather, Belmont-type frameworks respond only to a limited set of ethical issues: harms or wrongs that result from discrete interactions between investigators and study participants in the course of research. The Belmont principles are unable address ethical problems that arise over time and at scale—patterns of harm in larger portfolios of interactions, resulting from the conduct of a range of agents that affects the functioning of important social institutions.
Current ethical frameworks for innovation governance face four challenges. First, such frameworks, because they focus on the responsibilities of individuals, struggle to address unfairness within social institutions and may exacerbate such unfairness. Yes, discrete actions may cause unfairness, but unfairness also accrues over time from patterns in the operation of institutions. Consider the workings of health care and public systems. Whether these systems can respond effectively, efficiently, and equitably to the needs of diverse populations is determined in part by long histories of inclusion and exclusion, including histories of neglect, indifference, oppression, and racism. The responsiveness of such systems is also profoundly influenced by choices concerning which research questions to pursue and how to allocate funding. But in an open society, individual researchers are permitted to make these sorts of choices, chasing down the questions that interest them. No individual researcher has the ability to rectify problems of exclusion and oppression long since built into health systems, or indeed other social institutions on which people rely.
The Belmont principles are insufficient to govern AI innovation because they do not specify the requirements of justice in a way that captures the role of social institutions and distributions of social labor in shaping innovation and its impacts.
Second, current ethical frameworks do little to ensure accountability among the full range of relevant stakeholders within the innovation ecosystem. Many critical decisions about what research to pursue, how to allocate funding, and whose needs will be the focus of innovation are made not by researchers subject to ethics oversight but by politicians, philanthropic officers, corporate executives, and leaders of government agencies such as the US National Institutes of Health. However, existing ethical frameworks rarely specify the ethical obligations of these government, civil society, and private-sector stakeholders. These frameworks, then, have scant influence over the individuals most empowered to decide whether innovation strengthens or undermines important social institutions, contributes to justice and equity, or exacerbates injustice and underserved inequalities.
Third, current ethical frameworks do a poor job addressing portfolio-level issues. These are ethical problems that arise across interrelated sets of decisions. There can be cases in which any given interaction in a portfolio of interactions, evaluated on its own merits according to Belmont principles, could be seen as ethically permissible even as the larger portfolio is morally problematic. In the case of biomedical research, for example, individual oncology trials might exhibit scientific and social value, but the portfolio of such trials might systematically favor the health needs of advantaged groups. The risk-benefit ratio of each of a dozen individual trials may appear reasonable, but the totality of these trials may expose participants to risks that could be avoided through coordination across studies. The same holds for decisions affecting the relevance and breadth of information produced from sets of studies, the degree to which sets of studies advance the pecuniary interests of firms rather than responding to the needs of clinicians and patients, and the extent to which firms offload risk onto consumers rather than addressing it during development. This shortcoming at the portfolio level is partly a function of the previous two dynamics. But it also derives from the myopic, case-by-case evaluation of study protocols and individual technologies.
Finally, most current frameworks struggle to address the problem of distributed responsibility, instead presuming a one-to-one correspondence between the actions of a party and the responsibility of that party for any morally problematic consequences of their actions. In contrast, a framework of justice recognizes the reality of a division of social labor, which distributes rights, prerogatives, and responsibilities across many parties, with consequences for responding to effects of innovation. Innovation produces a social dynamic in which one era’s technological marvel, such as the telegraph or the steam engine, is eclipsed by subsequent advances. The displacement of legacy technology often produces unemployment, which in turn reduces the freedom of displaced workers. That displaced workers deserve support and assistance in transitioning to new forms of gainful employment is widely understood as a social obligation that falls to institutions of government and civil society and is not the sole responsibility of the innovators whose advances cause the decline of preexisting industries.
Each of the above challenges is relevant to issues of fairness in machine learning and AI. The AI ethics literature tends to conceptualize fairness as equal treatment relative to local norms for the distribution of a good or service and to focus on mitigation measures that target the statistical model employed by a given system. This approach, like the ethics review of individual research protocols, assumes that societies are patchworks of independent interactions, whereas in fact societies are interconnected systems in which social institutions affect overlapping aspects of people’s opportunities, capabilities, rights, and interests. As a result, unjust disparities and social inequalities arising from histories of oppression can be perpetuated by the enforcement of local fairness norms. The reason is that prior injustice in one domain creates disadvantage that can affect the prospects of the disadvantaged in other domains. Consider how past and present injustices in housing, finance, or policing can have profound detrimental impact on the health of oppressed populations, the quality of education available to them, their ability to take advantage of educational opportunities, their career prospects, their ability to vote or hold political office, their freedom to move about and associate, their financial prospects, and other important rights and interests. There need be no violation of local fairness norms in, say, schooling in order for injustices in housing to translate into worse outcomes in education.
The AI ethics literature tends to conceptualize fairness as equal treatment relative to local norms for the distribution of a good or service and to focus on mitigation measures that target the statistical model employed by a given system.
In such cases, there may not be a one-to-one correspondence between the actions of particular individuals, unjust outcomes, and responsibility for ameliorating unfair disadvantage. As a result, a narrow focus on local norms of fairness in discrete transactions cannot discern larger patterns of injustice across portfolios of interactions, cannot facilitate the process of ascertaining how to intervene to improve matters, and cannot help to identify who should be responsible for doing so.
Toward a Justice-Led Approach
Effective governance of innovation, in AI and other areas, requires that we broaden the set of stakeholders whose conduct is subject to accountability as well as oversight and intervention by institutions committed to a framework of justice. That framework must be substantive enough to generate normative guidance while also being widely acceptable to individuals who embrace diverse conceptions of the good and the good life. My book For the Common Good: Philosophical Foundations of Research Ethics covers this sort of framework in detail. Here, I’ll introduce three key elements of my proposed justice-led approach to innovation.
First, justice should be understood as fundamentally concerned with establishing, fostering, or restoring the freedom and moral equality of persons. Second, to respect people as free and equal requires specification of the space in which individuals are equal and in which they have a claim to equal treatment. In a diverse, open society, individuals embrace and follow different conceptions of the good. These different conceptions of the good often include competing hierarchies of value, divergent senses of worth, and inconsistent lists of virtues and vices. But amidst this diversity, every individual shares a higher-order interest in having the real freedom to formulate, pursue, and revise a life plan based on their considered conception of the good. This higher-order interest constitutes a compelling ground for claims of equal standing and equal regard because it is universally shared and because, from this higher-order perspective, there are no grounds on which to deem any individual, or set of individuals, superior to or more deserving than any other. That is, relative to their universally shared interest in having real freedom to formulate and pursue their favored conception of the good—their life plan—all persons are morally equal.
Third, justice is fundamentally concerned with the operation of basic social institutions—basic in the sense that they structure the division of social labor, distribute important rights and opportunities, and operate in a way that determines whether individuals have the real freedom to formulate, pursue, and revise a life plan of their own. Basic institutions include the organs of national, state, and local government because these determine the mechanics of political representation, make and enforce laws, and set the terms on which individuals access all-purpose goods such as employment, education, and the fruits of scientific progress in the areas of individual and public health. Basic institutions also include a network of private organizations that perform socially important tasks such as delivering health care, providing legal services, engaging in scientific inquiry, delivering education, and providing services in the market.
Effective governance of innovation, in AI and other areas, requires that we broaden the set of stakeholders whose conduct is subject to accountability as well as oversight and intervention by institutions committed to a framework of justice.
These three elements of justice can provide normative guidance aimed at rectifying the effects of prior injustice and ensuring that basic institutions function fairly and effectively, even in the face of technological change. To be clear, it is impossible to guarantee that innovation never disadvantages anyone relative to their considered life plan, but we can advance justice by safeguarding the ability of basic social institutions to create conditions that enable all persons to develop and exercise the capabilities they need to formulate, pursue, and revise a life plan of their own.
One significant feature of a justice-led approach to governing innovation is the establishment of incentives that encourage technological advance in service of people’s capacities to secure their shared, higher-order interest in freedom and moral equality. In particular, incentives should be used to align the interests of a wide range of parties with the goal of enhancing the ability of social institutions to maintain or promote freedom and equality. For instance, market forces alone are unlikely to incentivize commercial entities to create AI medical tools that address the distinctive health needs of people historically underserved by the health care system. Promoting a more equitable distribution of the benefits advanced by health care innovation will require a mix of approaches that reward this type of distribution while discouraging practices that increase disparities. In general, identifying gaps in the capacities of social institutions is a first, critical step toward adjusting funding priorities, regulatory requirements, and other incentives to better align the narrow interests of public and private stakeholders with opportunities to secure the freedom and equality of persons.
Furthermore, when innovation threatens the capacity of social institutions to effectively, efficiently, or equitably perform their unique functions, justice requires that there be intervention to strengthen those institutions. For example, the proliferation of machine learning and AI systems raises concerns about justice because the data on which these systems are built—and therefore the functions they perform—often reflect patterns of unfair treatment, oppression, marginalization, and exclusion. Algorithms used in policing, sentencing and bail calculations, or parole decisions that recapitulate histories of exclusion or oppression are unjust because of the strong claim of each individual to equal standing and equal regard before criminal justice institutions. The same is true of disparities fostered by AI systems that make decisions regarding employment, lending, banking, and the provision of social services. Such disparities are unjust even if they are not connected to prior histories of exclusion, indifference, or subjugation, because of the important roles these social institutions play in securing persons’ higher-order interest in freedom. But such disparities can be, and often are, doubly concerning precisely because they are connected to, and do recapitulate or compound, histories of subjugation.
Identifying gaps in the capacities of social institutions is a first, critical step toward adjusting funding priorities, regulatory requirements, and other incentives to better align the narrow interests of public and private stakeholders with opportunities to secure the freedom and equality of persons.
When innovation creates or exacerbates inequalities in the ability of individuals to advance their shared higher-order interest—when it promotes the freedom of individuals seen as normal and restricts the freedom of individuals seen as lesser on the basis of features such as sex, race, or creed—then social institutions should intervene to avert and rectify these undeserved inequalities. Such inequalities are, unfortunately, embedded in deployments of AI today. The widespread use of data in which marginalized groups are not well-represented, or are represented in ways that are associated with negative stereotypes or valuations, recapitulates patterns of subordination. And even when innovative technologies do primarily support individuals’ pursuits of their distinctive life plans, concerns of justice arise to the extent that patterns in the performance of these technologies recapitulate historical disparities. Widespread acceptance of these disparities signals that some individuals have lower standing or status than others—a message that is antithetical to justice. When social institutions act to reduce these disparities, they advance an important cause of justice: ensuring that all people are treated as free and equal.
These elements of a justice-led approach help to address portfolio-level issues. When we place justice first, we augment our focus on discrete interactions among a narrow set of stakeholders. We focus as well on broad patterns that emerge over time within larger sets of decisions and on the effects of strategies for dividing social labor among a wide range of interested parties. Relatedly, this justice-led approach can promote accountability among the full range of relevant stakeholders and address the problem of distributed responsibility. That is because this justice-led approach attends to the functioning of basic social institutions whose role is precisely to secure the freedom and equality of persons, regardless of the sources of injustice. Because the free and equal status of technology developers, scientific researchers, corporate leaders, government officials, and other stakeholders must also be respected, a central tool for advancing accountability and freedom is the construction of incentives designed to better align their parochial interests with the ends of justice.
This discussion only sketches the work required of us, but it points to a perspective that is sensitive to the broad range of growing concerns about the social impact of AI systems. Already AI technologies are proliferating in ways that threaten the ability of citizens to hold political leaders accountable, to distinguish truth from fabrication, to ensure the integrity of elections, and to participate in democratic deliberation. These threats, alongside the discriminatory outputs of some AI systems now on the market, implicate matters of justice. And so it is through the pursuit of justice that we may also head off these threats, governing the use of AI in the interest of the common good.